3. The Role of Theory in Research
3.4. Evaluating Theories
Victor Tan Chen; Gabriela León-Pérez; Julie Honnold; and Volkan Aytar
Learning Objectives
- Identify criteria for assessing whether a theory is good or bad.
- Understand the conditions that have to be in place for a relationship to be causal.
To recap what we’ve covered so far, using theories in research has many benefits. First, theories can tell us how, why, when, and where a particular phenomenon occurs. They explain the underlying logic and processes (what we’ve called mechanisms) that drive the appearance of that phenomenon. They tell us where and when we might expect to see it, and what outcomes and consequences it has.
Theories also help us make sense of the existing research on a particular topic. A good theory can inspire us to see existing theories in a new light. We can place past studies within a single theoretical framework (a collection of theories and related empirical observations). This lets us better see how everything fits together. It gives us insights into the significance and underlying assumptions of past research. And it guides future research, by helping us to identify concepts and relationships that are worthy of further study. As we gather more data, we can reconcile any contradictory findings by expanding or refining existing theories to account for them. We can also develop new theories to bridge gaps between existing ones—even between sociology and other disciplines.
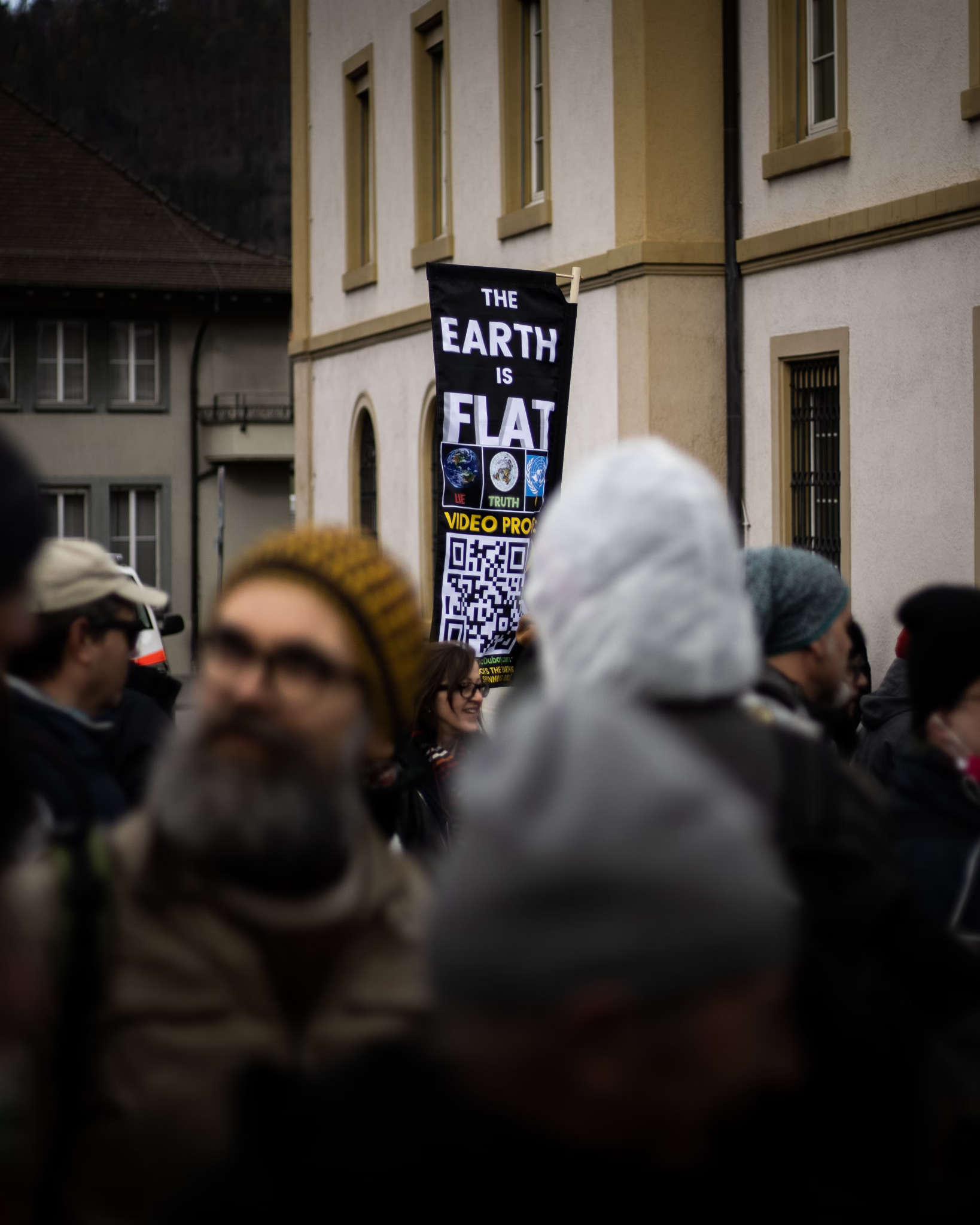
That said, it is important for researchers to recognize that the explanations their theories provide may be good or poor. There is nothing sacrosanct about any theory, and theories should not be accepted just because they were proposed by someone well-known and respected. Indeed, science makes progress when scientists see that a theory falls short in a particular area, and then refine or rework the theory so that it better describes, explains, and predicts reality. In the course of this work, poorer theories are eventually replaced by better theories with higher explanatory power. The essential challenge for researchers is to build better and more comprehensive theories that can explain a phenomenon more accurately and comprehensively than prior ones.
We should also keep in mind that every theory is incomplete—they are by definition simplifications of reality. Especially if we want them to help guide our decisions, theories have to reduce a phenomenon to a limited set of concepts and relationships. As social scientists, we’re always balancing the need between making our theories simple enough that they are understandable and actionable, and making them sophisticated enough that they accurately depict the world around us. Furthermore, scholars naturally tend to gravitate toward certain theoretical perspectives (more about that later), which may impose blinders and limit their sociological imagination. Seeing the world from a particular vantage point, they may miss out on important concepts that are not adequately addressed by their pet theory. We should keep in mind that theories—even our favorite ones—will rarely provide a full or utterly convincing explanation for a given phenomenon. Social life is too complex for that to be the case.
So, how can we evaluate theories? Here are some common criteria for judging whether a given theory is “good” or “poor”:
- Logical consistency: Are the theory’s concepts, hypotheses, and scope conditions consistent with each other? Any contradictions might mean the underlying logic of the theory is flawed. For example, suppose a theory assumes that people are rational actors—making logical decisions based on the costs and benefits of any actions. If we are studying situations in which people tend to make nonrational decisions—based on emotion rather than reason, for instance—the theory will probably not be a convincing explanation of people’s behavior within these scenarios.
- Explanatory power: How much does a given theory explain (or predict) reality? Good theories obviously explain the target phenomenon better than rival theories. For example, if one theory argues that a college degree pays off because educated people are smarter workers, and another theory argues that employers just pay people more who have that credential, then one of these theories should be able to account for more of the pay bump that college graduates receive than the other theory.
- Falsifiability: The British philosopher Karl Popper once said that for theories to be valid, they must be falsifiable. In other words, it must be possible to prove that a hypothesis generated from the theory is untrue based on empirical observations. Tautological statements, such as “a day with high temperatures is a hot day,” are circular in their reasoning; they are not empirically testable because a hot day is defined (and measured) as a day with high temperatures. As a result, such statements cannot serve as hypotheses, and the underlying theory is not falsifiable. Furthermore, whenever we evaluate a theory, there should be rival (alternative) explanations for the phenomenon in question. When we were discussing the payoff to a college degree earlier, we noted such competing explanations: one being that employers pay college graduates more for their actual skills, and the other being that they pay more for a “signal” of that skill—the college degree. We can decide between these two theories—and falsify one of them—by, for instance, seeing how much people with the same level of skill but differing credentials are paid. (As it turns out, there is a large bump in pay between people with almost enough credits to graduate college and those who do graduate—which suggests that it’s really the signal that employers focus on.) Saying that a theory is falsifiable, however, is not the same as saying that a theory should be falsified—you have better things to spend your time on than proposing a theory that you think will turn out to be false. Rather, the important thing is that your theory can possibly be proven false. Otherwise, there’s no point in conducting research to test that theory—your study already has a big thumb on the scale in its favor.
- Parsimony: Parsimony refers to how economical a theory is—how much of a particular phenomenon it can explain, with how few variables. The fourteenth-century English logician William of Ockham (also written as “Occam”) developed a principle for making theories parsimonious, which is called “Occam’s razor” in his honor. According to this principle, of all the competing explanations that explain the observed evidence, the simplest theory—the one that uses the fewest variables or makes the fewest assumptions—is the best. After all, our ability to explain a complex social phenomenon can always be enhanced by adding more and more concepts. Such an approach, however, defeats the purpose of having theories, which, as we noted earlier, are meant to be simplified explanations of reality. Parsimonious theories can be more easily generalized to other contexts, settings, and populations, and we should favor them if their explanatory power is the same as that of more complex theories.
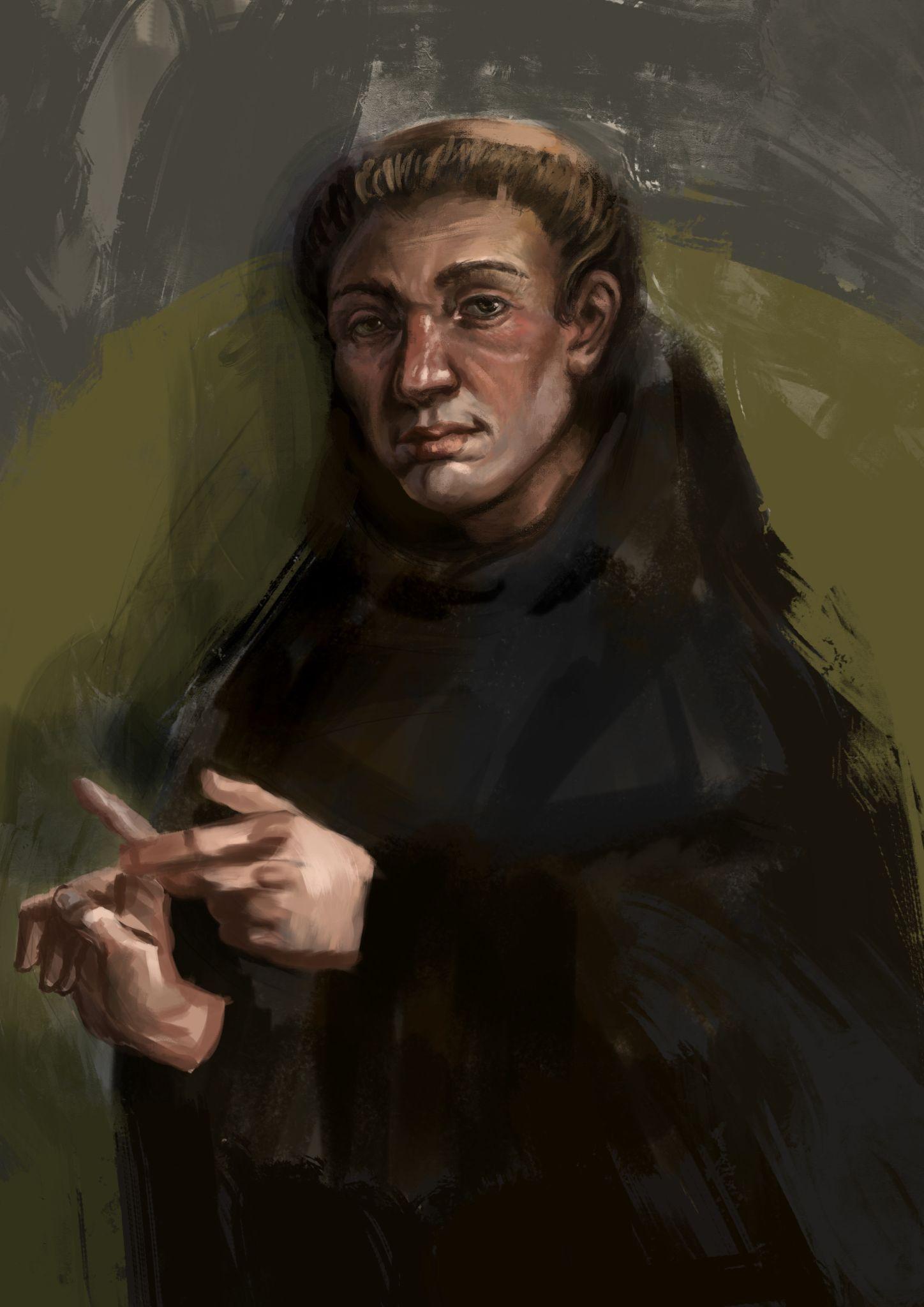
The second item, explanatory power, merits further discussion, given how crucial it is to identify and understand cause-and-effect relationships in the social sciences. Here are four criteria for a good causal theory:
- Correlation: A change in one variable is associated with a change in the other. (This is the bare minimum requirement for causality—remember, “correlation is not causation”!)
- Temporal precedence: The cause occurs before the effect. Here, we’re interested in the sequence of events: the change in the independent variable has to take place before the change in the dependent variable, or the former cannot logically be considered a cause of the latter.
- Rejection of alternative hypotheses: After testing whether other variables explain the phenomenon of interest, we conclude that these other possibilities are less convincing.
- Causal mechanisms: We can describe the specific process by which changes in the independent variable lead to changes in the dependent variable.
Some researchers might just stop at the first step—they care about correlations, and not about whether a change in one variable truly causes a change in another. Predicting events or behaviors using a set of variables can be useful in itself, even if you can’t necessarily explain why those events or behaviors are taking place. For example, market analysts routinely predict fluctuations in the stock market based on market announcements, earnings reports of major companies, and new data from the U.S. Federal Reserve or similar government agencies. Past research has identified correlations between those regularly observed variables (what are called indicators) and the overall performance of the market. Based on those past associations, analysts can plug in the current values of the relevant variables into their statistical models and predict what the market will look like at some later point. The same goes for the results of future elections: social scientists often use a mix of political polls, economic indicators, and other measures of voter mood to predict who will win elections—sometimes far into the future. The relationships here are not necessarily causal, but prediction requires us only to measure correlation. Indeed, the models we use in these cases may be rather stripped down—as we have described, models are always useful simplifications—with just some “inputs” (the values of our independent variables) that the model somehow converts into “outputs” (the predicted values of our dependent variable).
As social scientists, however, we usually want to do more than just observe associations between variables. We want to explain them. We want to understand how exactly a change in one variable leads, logically, to a change in the other. Scientists want to peer inside the “black box” that connects the input and the output—the independent variable and the dependent variable—and comprehend what’s going on there. That’s what scientific knowledge is about.
Key Takeaways
- Good theories are logically consistent, falsifiable, and parsimonious explanations of the relationships between concepts.
- To show that a relationship between two concepts is causal, the researcher must go much further than simply identifying a correlation. The change in the independent variable must precede the change in the dependent variable, and any alternative explanations must be ruled out. A good causal explanation will also have concrete causal mechanisms in mind.
The possibility that empirical observations can show a hypothesis generated from a theory to be untrue. The British philosopher Karl Popper argued that falsifiability is a foundation of the scientific method: for theories to be valid, they must be falsifiable.
How economical a theory is, as measured by how much of a particular phenomenon it can explain, with how few variables.
Another term for variables—operational definitions of concepts—that is frequently used in quantitative research, especially for variables that are routinely collected by government agencies and other organizations.