7. Measuring the Social World
7.4. Measurement Quality
Victor Tan Chen; Gabriela León-Pérez; Julie Honnold; and Volkan Aytar
Learning Objectives
- Define reliability and validity and discuss how they are related to one another.
- Describe the three key types of reliability and five key types of validity.
- Discuss how measurement quality in qualitative research is assessed differently than in quantitative research.
Once we’ve managed to define our terms and specify the operations for measuring them, how do we know that our measures are any good? Without some assurance of the quality of our measures, we cannot be certain that our findings mean what we think they mean. When social scientists measure concepts, they aim to achieve reliability and validity in their measures. Reliability concerns the consistency of our measures. A measure is said to be reliable if it gives the same result when applied repeatedly to the same phenomenon. Validity is about a measure’s accuracy. A valid measure truthfully reflects the meaning of the concept under study. These two criteria are central to how we evaluate the soundness of measures in quantitative research. As we will discuss later, they can be applied to qualitative research as well, although qualitative sociologists tend to favor other criteria when judging the rigor of their work.
Reliability: A Measure’s Consistency
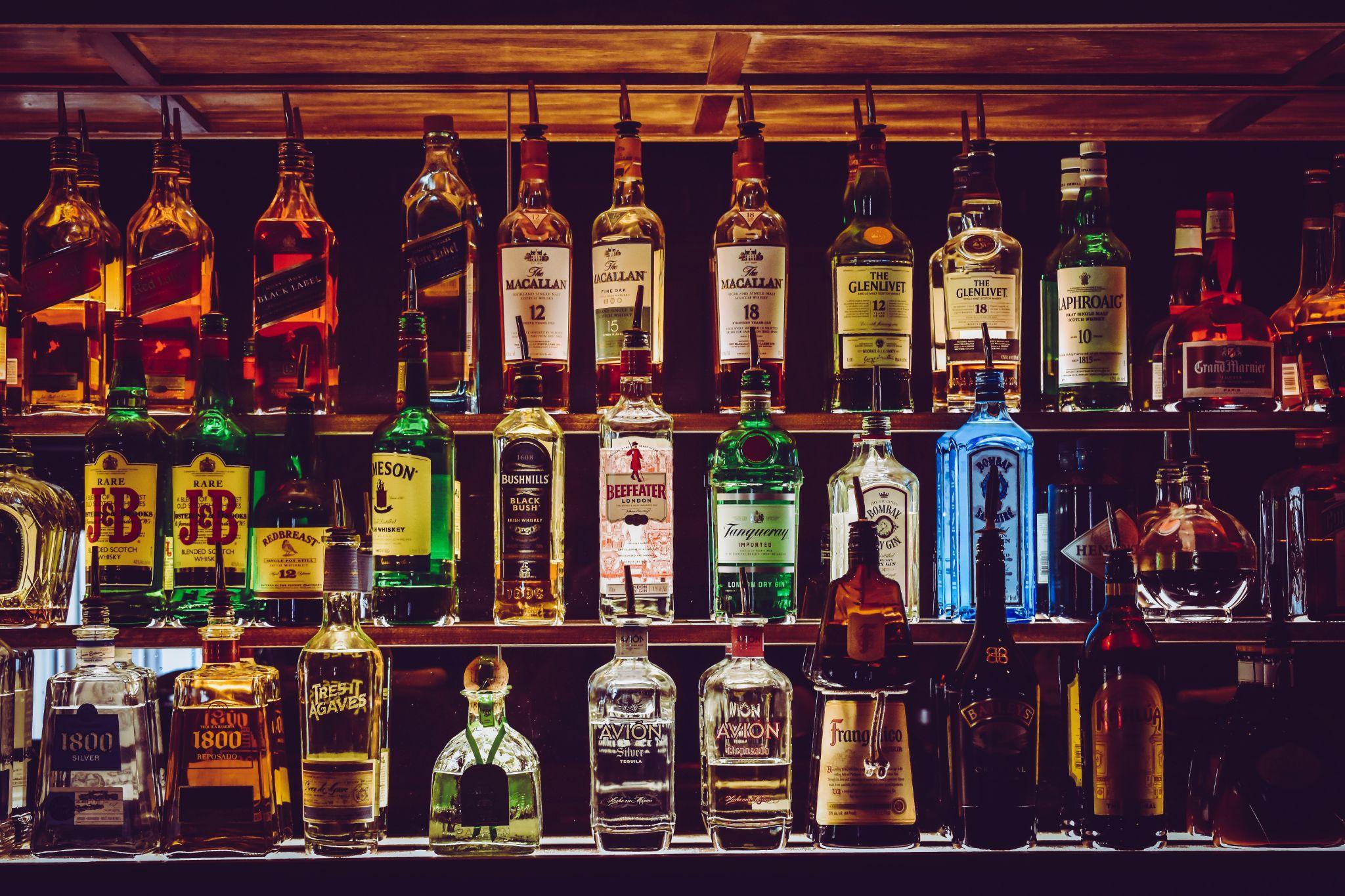
Let’s say we want to administer a survey about alcoholism and alcohol intake. We decide to measure alcoholism by asking people the following question: “Have you ever had a problem with alcohol?” If we measure alcoholism in this way, it seems likely that anyone who identifies as an alcoholic would answer this question with a “yes.” So, this must be a good way to identify our group of interest, right? Well, maybe. Think about how you or others you know might respond to this question. Would your responses after a wild night out partying differ from what they would have been the day before? Or, suppose you are an infrequent drinker, and the night before you had a single glass of wine—which gave you a headache on the day you’re taking the survey. Would that change how you might answer the question? In each of these cases, it’s possible that the same person would respond differently at different points in time. This suggests that our measure of alcoholism has a reliability problem.
Memory often creates issues with a measure’s reliability. If we ask research participants to recall some aspect of their past behavior, we should try to make the recollection process as simple and straightforward for them as possible. For example, if we ask respondents how much wine, beer, and liquor they’ve consumed each day over the course of the past three months, how likely are we to get accurate responses? Unless a person diligently keeps a journal documenting their intake, they will likely give us answers to that question that are all over the map—maybe accurate, but maybe wildly inaccurate. In contrast, if we ask the respondent how many drinks of any kind they have consumed in the past week, we should consistently get more accurate responses. People will be better able to remember what happened over a week’s time. As a result, the second question is a more reliable measure.
Reliability can be an issue even when we’re not reliant on others to accurately report their behaviors. Perhaps you are interested in observing how alcohol intake influences interactions in public locations. You may decide to conduct observations at a local bar, noting how many drinks patrons consume and how their behavior changes as their intake changes. But what if you go to the restroom and miss the three shots of tequila that the person next to you downs during the brief period you are away? The reliability of your measure of alcohol intake—counting the numbers of drinks that patrons consume—depends on your ability to actually witness every time that a patron consumes a drink. If you are unlikely to be able to observe every such instance, then perhaps your procedure for measuring this concept is not reliable.
When designing a study, you should keep in mind three key types of reliability. First, multiple applications of a measure over time should yield consistent results (assuming that the phenomenon being measured has not changed). For example, if you took standardized tests to measure your college readiness on multiple occasions in your senior year of high school, your scores should be relatively close from test to test. This is known as test-retest reliability. In the same way, if a person is clinically depressed, a depression scale should give results today that are similar (although not necessarily identical) to what it gives two days from now. In both these cases, we do not have reason to believe that the phenomenon being measured—scholastic aptitude or clinical depression, respectively—has changed in any substantial way, so the results across those applications should be similar.
If your study involves observing people’s behaviors—for example, watching sessions of mothers playing with infants—you may also need to assess inter-rater reliability. Inter-rater reliability is the degree to which different observers agree on what happened. If you and another researcher both watched a parent interacting with their child, would the two of you evaluate the parent’s engagement in a similar way? If your scores varied wildly, we might suspect that how you are measuring parental interactions is unreliable. Perhaps you identified certain behaviors as signs of an engaged parent that your fellow researcher did not. Or perhaps you are a keenly observant person, and you caught moments that the other researcher missed—such as when the infant offered an object to the mother, and the mother dismissed it. A similar issue arises if you are reading through interview transcripts or conducting a content analysis. Your interpretation of what is being said or what themes arise in your data may differ dramatically from another person’s version.
A research team can reduce these problems of inter-rater reliability by establishing clear and well-enforced standards for conducting observations and categorizing the data. They may write a formal document with guidance and examples regarding how to evaluate certain situations or outcomes. When making sense of a particular piece of data, such as an interview transcript, they may have two or more people review the same data to see if there are major differences in the conclusions they individually draw; if so, more training, documentation, and discussion could bring people’s perspectives into closer alignment.
With such safeguards, the scores of different observers should be consistent, although perhaps not perfectly identical. If a single person is doing all the observations for a study, however, there’s no way of checking whether that person’s observations would diverge significantly from another person’s. If possible, you should measure inter-rater reliability during pilot testing—enlisting colleagues to help you as needed—so that you can put procedures in place to narrow any such gaps. For published work, research teams are often required to include a measure of inter-rater reliability in their write-up to ensure that they are upfront about any problems in this area. Even solo researchers must acknowledge potential problems and defend their analysis from charges that it is fundamentally unreliable.
When crafting multiple-item measures, internal consistency is a third important thing to consider. The scores on each question of an index or scale should be correlated with each other, as they all measure aspects of the same overall concept. Let’s say we created an index that measures support for feminist issues. It includes questions about whether the person would vote for a woman for president and whether they support paid family leave. If we ask a group of people these questions, we would expect to find a strong correlation between the scores for each question. A person who would vote for a woman for president would probably also express support for paid family leave, for instance. The two questions don’t capture the exact same thing, but if both are feminist issues, they should be related in terms of how people answer them. This should be the case for all the questions in the index or scale if it is internally consistent. (As we will discuss further in Chapter 14: Quantitative Data Analysis, a specific statistical test known as Cronbach’s alpha—written as α—provides a way to measure how closely answers to each question in an index are related to those for the other questions; you will frequently see this figure reported in papers that use indexes or scales.)
Ideally, you should evaluate these three types of reliability—test-retest, inter-rater, and internal consistency—both in your study’s pilot-testing process and after your data collection is done. That said, the design of your study will affect your ability to do so. For example, if you are conducting a longitudinal study that involves collecting data from the same participants at multiple times, you can evaluate test-retest reliability. You won’t be able to do so, however, in a cross-sectional study after the data collection is complete.
Validity: A Measure’s Accuracy
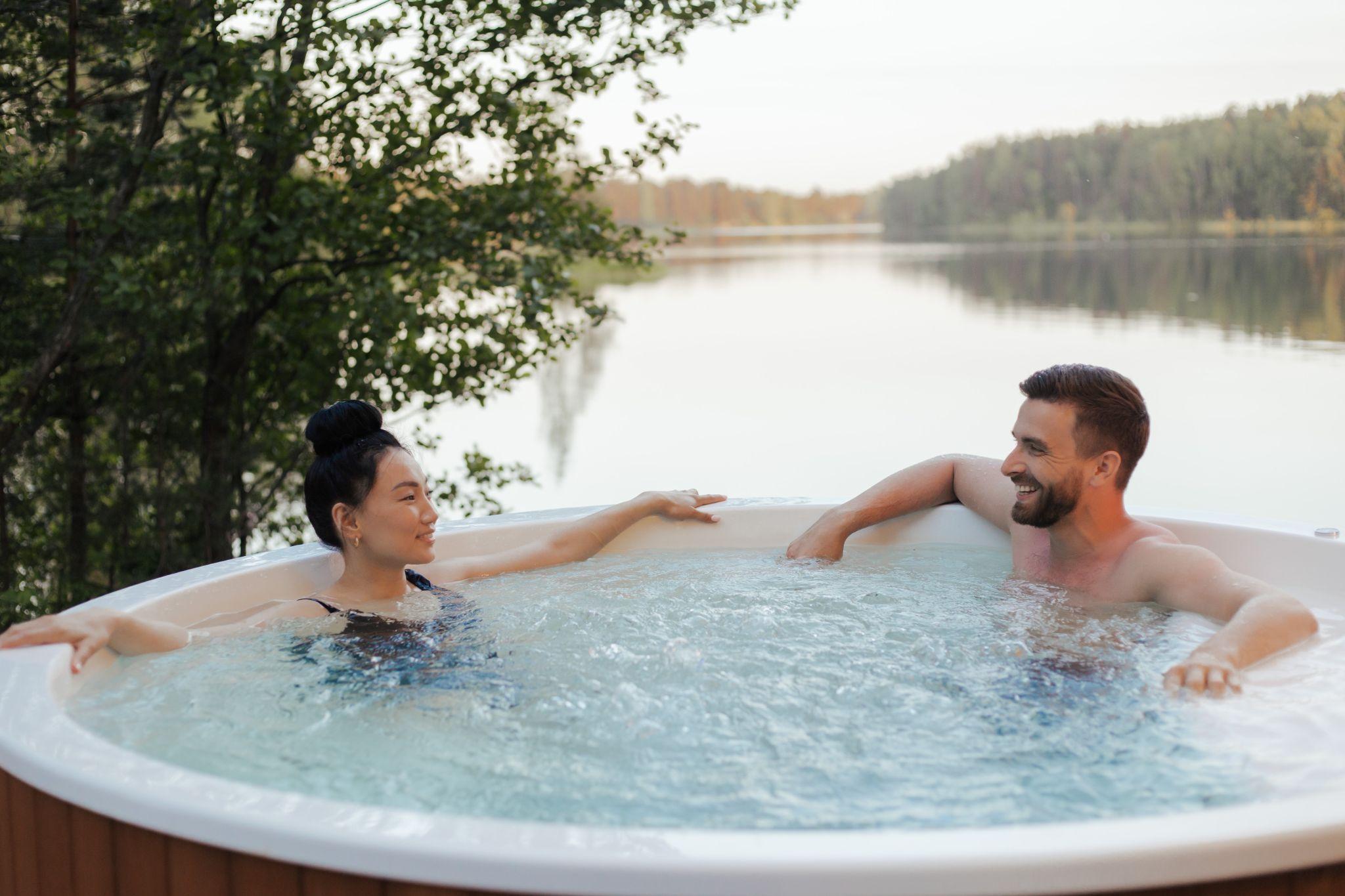
While reliability is about whether a measurement procedure gives consistent results, validity (also known as construct validity) is about whether the procedure actually measures the concept it is intended to measure. Put a bit differently, valid measures accurately get at the meaning of our concepts. Think back to the measures of alcoholism we considered earlier. We initially considered asking research participants the following question: “Have you ever had a problem with alcohol?” We realized that this might not be the most reliable way of measuring alcoholism because the same person’s response might vary dramatically depending on how they are feeling that day. But this measure of alcoholism is not particularly valid, either. For one thing, it’s unclear what we mean by “a problem” with alcohol. Some people might think they have “a problem” after experiencing a single regrettable or embarrassing moment after consuming too much. For others, the threshold for “a problem” might be different: perhaps a person has had numerous embarrassing drunken moments but still gets out of bed for work every day, so they don’t perceive themselves as having a problem.
Because what each respondent considers to be problematic might be very different, our measure of alcoholism isn’t likely to yield any useful or meaningful results if our aim is to objectively understand, say, how many of our research participants are alcoholics. (Granted, the definition of alcoholism is partly subjective and open to debate, but this particular question remains a poor measure of whether the person is an alcoholic based on any common understanding of the term.) Note that the problem isn’t the fact that the same person will respond to the question differently at different times—a problem of reliability—but that different people will interpret the question in different ways—a problem of validity.
There are different ways of evaluating whether a given measure is valid. When we were arguing that the question “Do you have a problem with alcohol?” is a flawed measure of alcoholism, we were using logical reasoning to assess its face validity. Face validity asks whether it is plausible that the question measures what it intends to measure. On the face of it, does the question pass muster? Logically speaking, can it measure what we want it to measure? Sometimes determining face validity is easy. For example, a question about a person’s place of residence would clearly lack face validity if we were using it as a measure of their political views; even if people’s politics often correlate strongly with where they live, we should really be asking them directly about their political opinions. Other times, however, face validity can be more difficult to assess. Let’s consider another example.
Suppose we’re interested in learning about a person’s dedication to “healthy lifestyle” practices. Most of us would probably agree that engaging in regular exercise is a sign of dedication to a healthy lifestyle, so we could measure this concept by counting the number of times per week that a person visits their local gym. One of the first things we’d have to consider is whether that operationalization has face validity. Does our operationalized variable measure the concept that it is intended to measure on the face of it? In the case of counting the number of times per week visiting a local gym, perhaps they want to use their tanning beds, flirt with potential dates, or sit for hours in the sauna. These activities, while potentially relaxing, are probably not the sort of “exercise” we had in mind as one dimension of a healthy lifestyle, and therefore, recording the number of times a person visits the gym may not be the most valid way to measure our “healthy lifestyle” concept on the face of it. Here, the problem isn’t that different people will interpret the same question differently, but rather that the question doesn’t quite measure what we want it to measure.
One method for assessing face validity is to pilot test measures with research participants who are like those who will be in the final study group. For example, if you were conducting a survey of transgender individuals about their access to appropriate healthcare services, you could attend transgender-related events and solicit a small number of volunteers to test your questionnaire. Following a common testing method, you could first have the pilot-testers answer the questions, and then ask them to identify any questions that were puzzling, ambiguous, or confusing. You could have the pilot-testers explain why they felt this way, thereby gaining some insight into how the survey questions might have faltered in measuring your study’s underlying concepts. For example, let’s say one of your questions was “Have you ever experienced discrimination by a doctor or other healthcare provider due to your transgender status or gender expression?” In your initial draft of that question, you only gave respondents the response categories “Yes” and “No.” In your pilot testing, however, your respondents mentioned that they did sometimes feel that providers discriminated against them, but they were not sure if it had anything to do with “transgender status or gender expression.” Based on this feedback, you ultimately decide to provide an “unsure” response option, ensuring face validity and thereby enhancing the overall validity of your measure.
To illustrate another type of measurement validity, let’s examine the sorts of operationalization decisions that might relate to our hypothetical study of transgender access to health care. For this study, we are interested in how transgender people learn about the healthcare services available to them. We decide to operationalize this concept—“sources of information about transgender health care”—by asking our respondents a question: “Have you received any information about transgender health care from your doctor?” If we think through the various ways that transgender people might learn about their healthcare options, however, we would soon realize that this single question is not enough: talking to their doctor is not the only way that our respondents could receive this sort of information. As a result, our single indicator does not cover all of the possible meanings of our concept.
Content validity assesses our measurement in this way: does our chosen measure get at all the important meanings or dimensions of our concept? “Sources of information about transgender health care” is a multidimensional concept that really needs an index or scale to measure it comprehensively. In addition to asking our respondents about whether they received any information from doctors, we need to ask about other possible sources, such as friends, transgender support groups, and the internet.
Always consider whether aspects of your concept of interest aren’t included in the particular measure you’re using to examine it. This is important regardless of whether you create the measure yourself or borrow it from previous research—in fact, one way that social scientists can contribute to the literature is by adapting past measures to current times (such as how social scientists have changed the measures they use to identify and count “families” in a given society, as we discussed in an earlier chapter). If you are unsure whether you are measuring all the relevant dimensions of a concept, you may need to do some more digging first—reading the relevant literature or conducting pilot interviews or focus groups with people who know that concept well (such as the transgender people in the previous example). Sometimes researchers will even convene panels of experts on the subject to reach some sort of consensus about what the different dimensions of a given phenomenon are and how best to measure them.
A third crucial kind of validity is predictive validity. Let’s say we have found an existing index that seems to measure the concept of “dedication to healthy living” in a rich and multifaceted way. To make sure our measure is truly valid, we don’t want to stop there—we should test how well it predicts relevant outcomes. For example, based on how our respondents score on our index of healthy living, we should be able to make good predictions of how well they will do on various health evaluations—say, how favorably the blood panel tests they take during their annual physicals turn out. If our measure is effective in this way, we say it has predictive validity: it predicts future phenomena that it should be able to predict, considering the underlying concept it is measuring.
Smart researchers are always on the lookout for ways to test the predictive validity of any measure that they or others choose to use. Sometimes, testing those predictions can become a matter of fierce debate. We already mentioned in the sidebar The Bell Curve and Its Critics how social scientists have challenged the utility of the IQ measure, and a similar controversy surrounds the SAT and similar standardized exams used as the basis for admissions decisions by colleges and universities. If SAT scores are a truly valid measure of “scholastic aptitude,” they should be able to predict student success in college—the higher a person’s score, the greater the likelihood they will do well in their college classes. Research on this question finds that high SAT scores are associated with high grades in college, but some social scientists argue that the SAT is nonetheless a flawed predictor that largely reflects the fact that kids from more advantaged backgrounds do well both on the SAT and in their college classes.[1]
Another good way to test a measure’s validity is to look for an existing measure of the same (or at least a similar) concept. You should use both measures when you collect your data and then analyze the results you get from each. If their scores are correlated, then you have reason to believe that both are measuring the same concept. This is called convergent validity: the two measures converge on a similar result. For example, if we wanted to evaluate our index of healthy living, we might turn to the Healthy Lifestyle Behaviors Scale (HLBS) (Walker, Sechrist, and Pender 1987). If we gave someone our healthy living index and the HLBS at the same time, their scores should be pretty similar.
Testing for discriminant validity uses the exact opposite approach. In this case, you compare your measure to a measure that, logically speaking, should be entirely unrelated. For example, our respondents’ scores on our healthy living index should not be strongly related to their scores on, say, a scale measuring the intensity of their political opinions. If they are related, then we have reason to question whether we’re really measuring what we intend to be measuring. Our assessment can be sharper if we compare our measure’s discriminant validity with its convergent validity. For example, we might reasonably expect not only that a measure of a person’s self-esteem will converge with a measure of their confidence in social interactions but also that the association we uncover will be stronger than the one between a person’s self-esteem and some clearly less relevant measure, such as their support of authoritarianism.
Thinking about Reliability and Validity in Research
We’ve touched upon many aspects of reliability and validity in the preceding sections, and by now you’ve probably mixed them up hopelessly in your heads. We’ve got you covered: Figure 7.7 illustrates a classic way of visualizing the differences between these two ideas.[2] Across these three targets, think of the placement of the shots as a sociologist’s attempts to measure a particular concept. The reliability of the measure—that it generates the same results under the same conditions—is represented by the consistency of the shots on the target: the tighter the pattern of shots, the higher the measure’s reliability. In the left-hand target, we see a wildly inconsistent pattern of shots—in other words, low reliability. In both the middle and right-hand targets, however, the shots are tightly clustered in a single area, signaling high reliability.
Now let’s consider the three targets in terms of validity. The closer the shots are to the bullseye—which represents the actual meaning of the concept—the higher their validity. In the left-hand target, the sociologist’s measure has a low level of validity: few of the shots are near the bullseye, meaning that the measurements do not accurately reflect the underlying concept. In the right-hand target, all the shots are on the bullseye. The measure is valid. The sociologist is measuring the right thing. Now, the middle target is particularly interesting. The measure being depicted clearly has high reliability, as all the shots are tightly clustered. Yet this measure would be unwise to use because its validity is low—it’s not measuring what the sociologist intends to measure. That speaks to an essential truth about research: there’s little use in being consistent if you are consistently wrong.
You also may be wondering why the diagram has only three of the four possible high-low combinations? Why didn’t we include a target illustrating low reliability and high validity? We left it out to make a point: it’s harder to hit a bullseye on a target (i.e., obtain highly valid measurements) when your shots are scattered (your measure has low reliability). A research project that gathers only unreliable measurements is unlikely to accurately capture its underlying concepts of interest and to generate valid results.
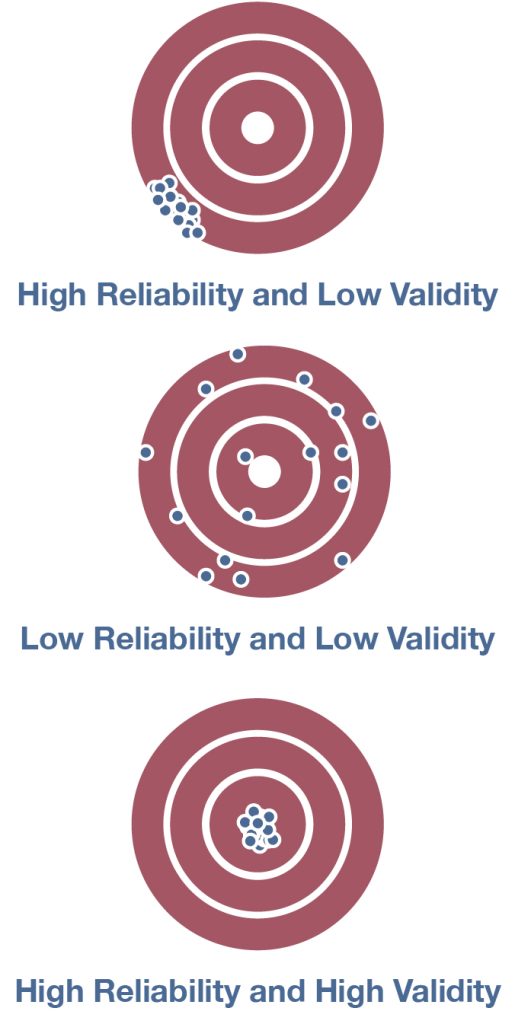
A final word of advice: as you read more sociological studies, you will find that there are many more ways to evaluate the reliability of measures rather than their validity. This is because assessing the consistency of a measure is generally more straightforward—as you can see in the simple procedures we just described. In contrast, social scientists engage in endless debates about whether a particular indicator or index is truly the best way of capturing the essence of a particular phenomenon. Just think about our earlier discussion of the numerous methods that scholars and governments use to measure poverty. This particular methodological debate is especially partisan and polarized, but you should be aware of how complicated an undertaking it is to arrive at a valid measure of many social concepts—even ones that aren’t as obviously political. You will have to be exceedingly careful in how you approach the steps of conceptualization, operationalization, and measurement we illustrated in Figure 7.1 to ensure that your study ultimately generates valid results. Among other things, you should pay attention to the process represented by the arrow curving back upward: always go back to the key concepts of your study and ensure that your measures truly reflect them.
Measurement Error: Random and Systematic
As we have described, good measures demonstrate validity and reliability. Nevertheless, when we actually take measurements out in the real world, they always have some degree of error. Measurement error is the difference between the values of a variable and the true—but unobserved—values of that same variable. For example, measurement error occurs when a person tells the interviewer her age is 46 when it’s actually 43, or when the U.S. Census Bureau counts the population of homeless people as lower than it actually is.
Measurement error can take two forms: random and systematic. Random error is present in any measurement. Even with the most precise instruments, our measurements are always a little bit off—never perfectly describing what really exists. That’s to be expected. If you’ve ever stepped on a bathroom scale twice and gotten two slightly different results—maybe a difference of a tenth of a pound—then you’ve experienced random error. Maybe you were standing slightly differently or had a fraction of your foot off of the scale the first time. If you were to take enough measures of your weight on the same scale, you’d be able to figure out (or at least get closer to) your “true” weight.
A similar kind of random error plagues our measurements in the social sciences. For example, if you gave someone a scale measuring depression on a day when they lost their job, they would likely score higher on it than on a day when they received a promotion. Even if the person was truly suffering from clinical depression, the random occurrences of that person’s life would alter our measures of that depression—much like a stray foot on the bathroom scale. For this reason, social scientists are taught to speak with humility about our measures. With all the factors at play when dealing with complex human beings, it’s exceedingly hard to reduce the random error that plagues social scientific measures. With enough rigor in our approach, we can be reasonably confident that what we have found is true, but we must always acknowledge that our measures are only an approximation of reality.
Although we should be resigned to some degree of random error in our measurements, systematic error is more worrisome. This issue arises when a measure consistently produces incorrect data, typically in one direction—making our measurements consistently different (say, too high or too low) compared with the true value. Imagine you surveyed students at your local college or university and asked them to give their height, but you didn’t provide any response categories for heights taller than six feet, six inches. Some of the taller members of the basketball team might not be measured accurately. The average height you calculate based on this data would not match the real average height of the student body—it would be lower by a certain amount, because the tallest people would have been miscategorized as shorter than they actually are.
Researchers can also create systematic errors in their measures when they use question wording that causes respondents to think one answer choice is preferable to another. For example, if an interviewer were to ask you, “Do you think global warming is caused by human activity?” you would probably feel comfortable answering honestly. But suppose the interviewer asked, “Do you agree with 99 percent of scientists that global warming is caused by human activity?” Would you feel comfortable saying no, if that’s what you honestly felt? Maybe not. That is an example of a leading question, a question with wording that encourages a respondent to answer in a certain way. (We discuss leading questions and other problems in question wording in greater detail in Chapter 13: Surveys.) Note that a poorly worded question sometimes creates confusion, but it does not prod respondents to answer in a particular way. In this case, the error is random, not systematic. We should be concerned that our measure is unreliable—people may answer very differently depending on how much they understood the question—but we don’t have reason to believe that the answers to our question are skewed in a particular direction. That’s not the case, unfortunately, for the sorts of leading questions we just mentioned, or for questions that suffer from other forms of bias—that is, factors that cause our study to give us a picture of social reality different from the true state of affairs.
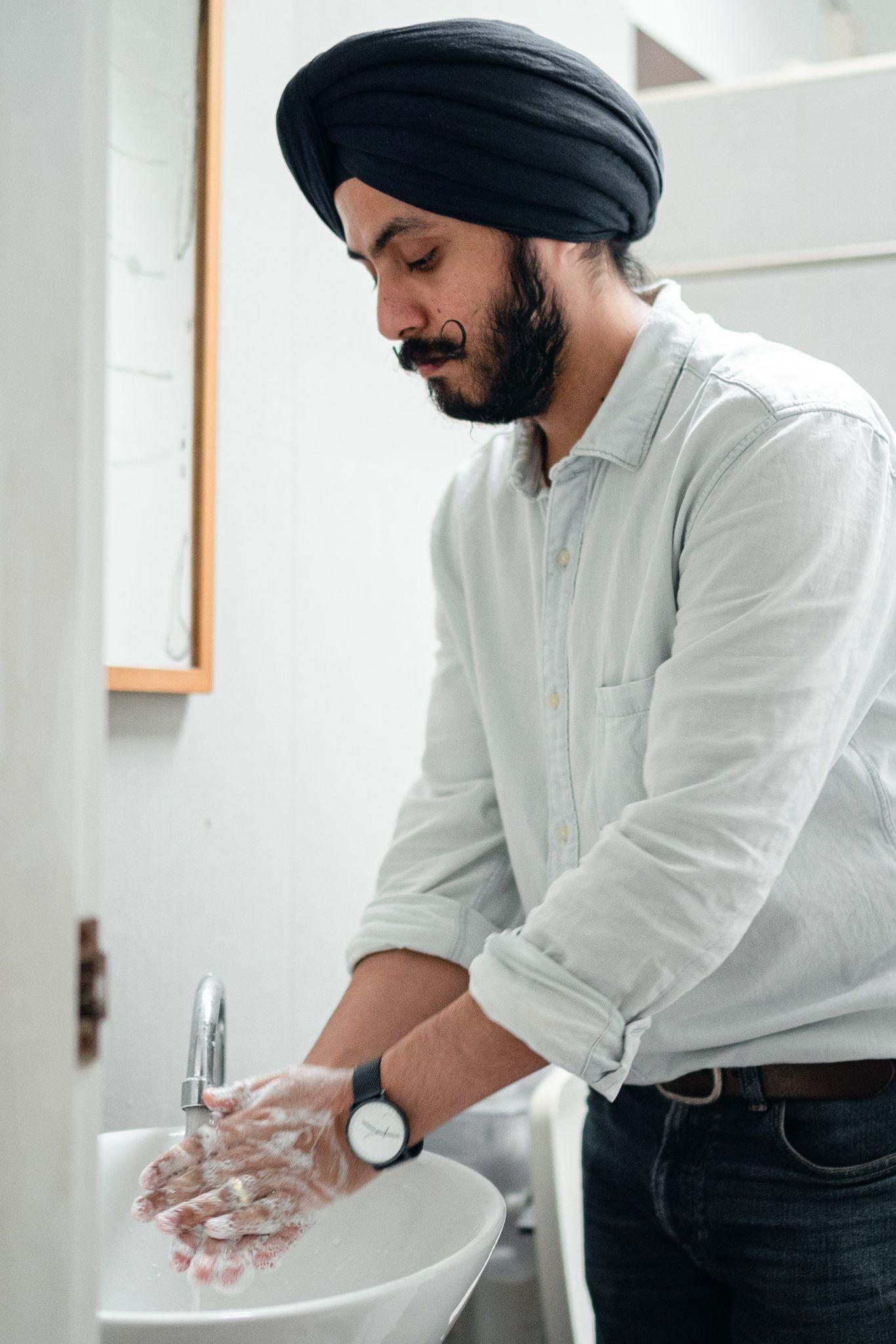
Imagine you were conducting a survey that asked people if they washed their hands after using the bathroom. Would you expect your respondents to be perfectly honest? Their answers might say more about what they would like others to think they do, rather than what they actually do. When participants in a research study answer or act in particular ways to present themselves to the researcher in a more positive light, we call this kind of bias social desirability bias. Generally speaking, people in any study will want to seem tolerant, open-minded, and intelligent, even if their true feelings are biased, closed-minded, and simple. This is a common concern in political polling. Pollsters are constantly worried about whether respondents are being honest about supporting a controversial candidate—or not supporting a third-party candidate, a person of color, or a female candidate. (See the sidebar Social Desirability in Political Polling.)
A related form of measurement error is called acquiescence bias, also known as “yea-saying.” It occurs when people say “yes” to whatever the researcher asks, even when doing so contradicts previous answers. For example, a person might say yes to both “I am a confident leader in group discussions” and “I feel anxious interacting in group discussions.” Those two responses are unlikely to both be true for the same person. Why would someone do this? One reason is social desirability: people want to be agreeable and nice to the researcher asking them questions. They might be trying to “save face” for themselves or the person asking the questions. Or, they might simply want to ignore their contradictory feelings when responding to each question. Regardless of the reason, the results of your measure in this particular situation will not match what the person truly feels. We could imagine a study in which more “yes” answers would support a particular finding, which would mean a large amount of systematic error that biases the study in the direction of that finding.
Usually, systematic errors will result in responses that are incorrect in one direction or another. For example, social desirability bias usually means more people will say they will vote for a third party in an election than actually do. Systematic errors of any kind can be a problem, but they are especially problematic when they skew our results in a particular direction—meaning that we grievously overstate or understate the prevalence of a phenomenon or the severity of a problem. Fortunately, we can take practical steps to reduce systematic errors. As we will discuss later in Chapter 13: Surveys, we can word questions in such a way to make it more likely that people will speak honestly. We can mix up our affirmative and negative questions so that acquiescence bias doesn’t push our study’s findings in one direction.
We can never eliminate random error, but by definition, this other type of measurement error is unpredictable and does not result in scores that are consistently higher or lower on a given measure. You can think of random error as statistical noise that you can expect to average out across participants. Like static in your headphones, random error may make it harder to make out certain things, but it won’t skew your statistics or your overall sense of the patterns in the data—which is a good thing.
Social Desirability in Political Polling: The Wilder Effect and Shy Trump Voters
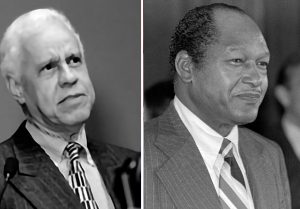
The 1989 election to decide Virginia’s governor pitted Democratic candidate L. Douglas Wilder against Republican candidate Marshall Coleman. Wilder was running to be the first African American elected governor of a U.S. state. Predictions from statewide polls during the campaign indicated that Wilder was heavily favored to win election. “Two weeks out from the race and maybe shortly before that, there were people proclaiming victory by saying that I was up by double digits,” Wilder recalled in an NPR interview decades later (Martin 2008). “Several polls said there was no way I could lose.” Wilder did indeed beat his white challenger—but he won by less than 1 percent of the vote. It turned out that both preelection political polls and election-day exit polls had wildly overestimated Wilder’s actual support among voters. The race ended up being so close that Wilder had to wait for a recount to confirm his win.
This was not the first time that political polls had blatantly overestimated an African American candidate’s support among the U.S. electorate. Tom Bradley, a former Los Angeles mayor, ran for governor of California in 1982. A poll taken about a month before the election had Bradley up by 22 points. Another poll conducted close to the election day had him with a 10-point lead. Bradley went on to lose in the closest election in California history (Payne 2010). After Bradley’s unexpected defeat, social scientists started talking about the “Bradley effect”—the notion that voters didn’t want to admit to pollsters that they weren’t actually going to vote for a nonwhite candidate. After Wilder’s near-loss, the term “Wilder effect” also came into circulation to describe this type of alleged polling bias.
As sociologists who conduct surveys know well, respondents will try to answer sensitive questions in a way that will present them in a favorable light. This so-called social desirability bias led to the flawed polling in California and Virginia, proponents of the Bradley/Wilder effect argued. The two men were running for political office during a period when voters were becoming increasingly reluctant to admit that they would not vote for a nonwhite candidate. Social desirability bias caused some respondents to tell pollsters they favored the African American candidate in these two races when they did not actually plan on voting for him on election day. The Bradley/Wilder effect has since become a popular theory in political circles. It remains controversial among social scientists, however, given the challenge of rigorously testing it. Muddying matters even further, the political scientist Dan Hopkins (2009) analyzed U.S. Senate and gubernatorial races from 1989 to 2006 and concluded that the Bradley/Wilder effect was present in political polls before 1996 but—for unclear reasons—vanished in later elections.
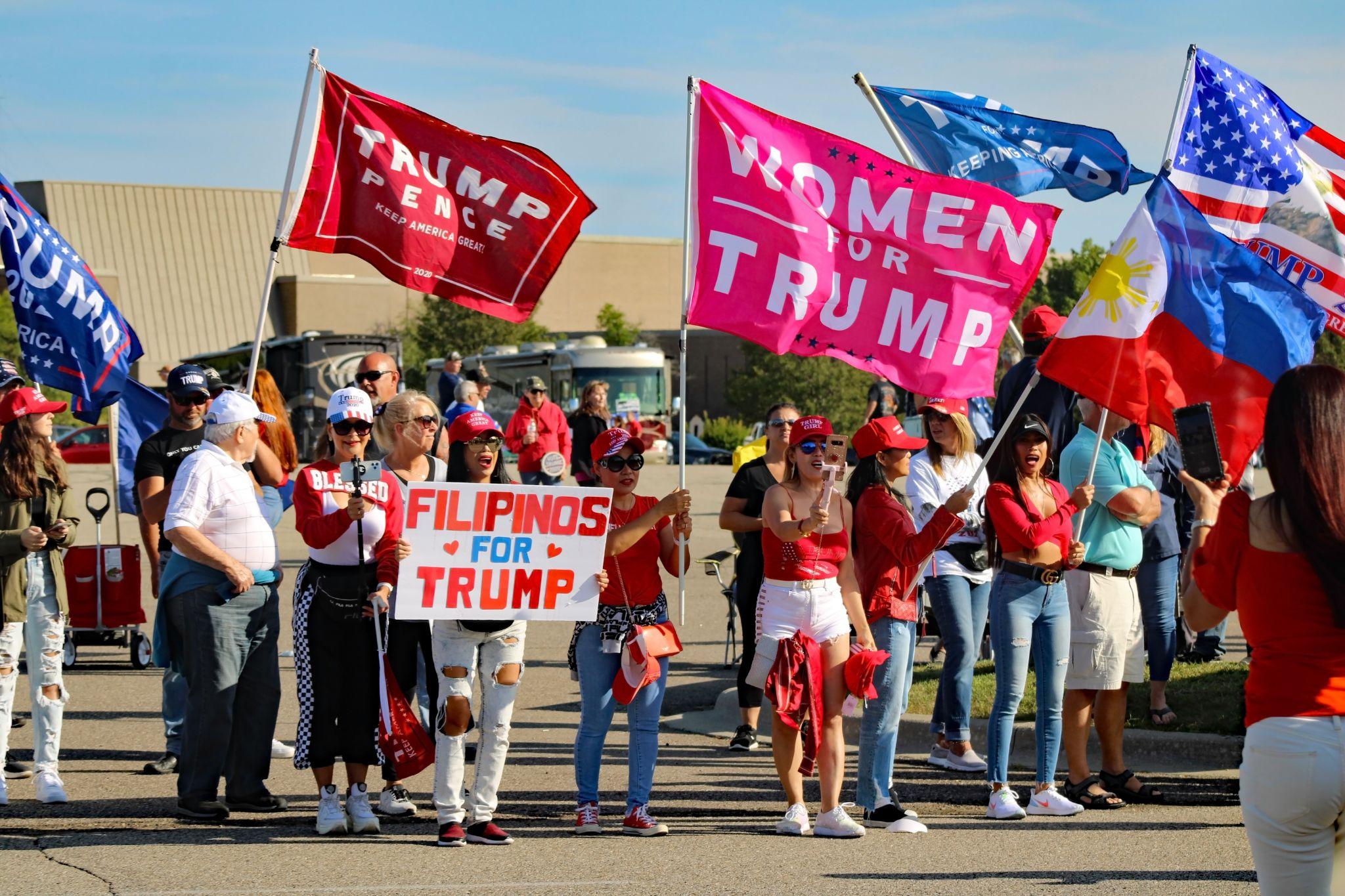
The surprising outcome of the 2016 presidential election—which, according to most preelection surveys, the Democratic candidate Hillary Clinton had been favored to win—generated new allegations that social desirability bias was once again skewing the polls. This time, political observers argued that the highly polarized attitudes about Republican Donald Trump’s campaign for president had made his supporters reluctant to admit they were planning to vote for him—or reluctant even to respond to pollsters from establishment organizations. These votes for Trump would not have been picked up by the preelection polls, which could explain why turnout for Trump ended up being much higher than predicted. As with previous electoral mispredictions, a compelling theory emerged after the 2016 election to explain the polling industry’s terrible, horrible, no good, very bad day. Despite all the airtime that this notion of “shy Trump voters” has sucked up on cable news in the years since, empirical support for it remains mixed (Coppock 2017; Enns, Lagodny, and Schuldt 2017).
If the size of these so-called Bradley/Wilder/Trump effects may be exaggerated, social scientists do worry a great deal about social desirability bias in their surveys—political polls or otherwise. There are some straightforward ways to reduce it (as we describe further in Chapter 13: Surveys), and more involved approaches can be undertaken when surveys need to ask about particularly sensitive topics. For example, survey methodologists have created “social desirability scales” to identify which respondents are more likely to offer up socially acceptable answers. Probably the most well-known is the Marlowe and Crowne Social Desirability scale (MCSD), developed in 1960 and still in use today, which contains four items in one condensed version of the scale (Haghighat 2007):
- Would you smile at people every time you meet them?
- Do you always practice what you preach to people?
- If you say to people that you will do something, do you always keep your promise no matter how inconvenient it may be?
- Would you ever lie to people?
The answers to these questions are used to generate an index score. The higher the score, the more likely respondents are to give socially desirable answers. The scale can be used to adjust the results of surveys—including preelection polls—to account for the fact that social desirability may be skewing expressed opinions upward or downward.
Social desirability bias is by no means the only reason that political polling may be wildly off in its electoral predictions. Far more important, as we discussed in Chapter 6: Sampling, is the selection bias that affects who actually is being represented in a poll’s sample. Furthermore, preelection polls by definition happen before election day, and political events can dramatically change a candidate’s level of support within a brief span of time—as arguably happened in the 2016 presidential election, with Clinton plagued by damaging news stories in the final days of her campaign.
Talking two decades later about his close gubernatorial race, Wilder himself speculated that the so-called Wilder effect wasn’t so much about voters reluctant to tell the truth but rather polls drawing too much from Democratic-leaning areas (Martin 2008). Remember that political polls are surveys, and surveys can fall short in their measurement and sampling for countless reasons.
Measurement Quality in Qualitative Research
In qualitative research, the standards for measurement quality differ in important ways from those for quantitative research. Data collection in quantitative research involves a level of objectivity and impartiality that qualitative research cannot match. In a straightforward fashion, quantitative researchers will choose a measure, apply it, and then analyze the results. As we have noted, however, qualitative researchers typically don’t ask the exact same questions of every respondent or conduct the exact same kind of observations in every space.
Rather than relying on fixed measurement instruments, qualitative researchers should see themselves, in effect, as their measurement instruments. What does this mean, in practice? For one thing, qualitative researchers must make full use of their rapport with research participants and their immersion in particular social spaces. This closeness to the data allows them, in turn, to present a rich picture of an individual or community’s lived experiences and subjective understandings and to describe in rich detail the social processes they observed. In the course of their fieldwork, qualitative researchers build connections between the different ideas that participants discuss. They ask follow-up questions or conduct additional observations to delve more deeply into how exactly a concept is understood or experienced. They then draft an analysis that accurately reflects the depth and complexity of what respondents have said or what they have witnessed.
This is a challenging set of tasks to do well. It requires balancing competing priorities. For instance, qualitative researchers always need to acknowledge their personal biases—either from their own experience or their existing knowledge of the topic—given that the very way they are gathering data makes it difficult to be truly objective and methodical (we will discuss the idea of positionality further in Chapter 9: Ethnography). At the same time, they have to be careful about accepting their respondents’ statements or perspectives uncritically. Qualitative sociologists carry a toolkit of useful theories and methodological skills into the field, but they also need to be open to the ways that the data they gather can contest or complicate the understandings they originally brought with them. In fact, this is often the analytical approach that qualitative researchers take in their fieldwork: not trying to prove or disprove a hypothesis, but rather looking for unexpected connections, complexities, or wrinkles in whatever theoretical perspective they’re using to view the world.
For these reasons, good qualitative research hinges not so much on being objective—there is always some subjectivity in qualitative analysis—but more on the degree of rigor that the individual researcher brings to bear in their fieldwork and analysis. The “rigor” we have in mind is not about validity and reliability per se. Although it is possible to critique qualitative research in these terms, many researchers use different language to describe the soundness of qualitative work. Two criteria often used are authenticity and trustworthiness (Rodwell 1998).
Authenticity refers to the degree to which qualitative researchers capture the multiple perspectives and values of participants in their study. While there are multiple dimensions of authenticity, the most important one for novice researchers to understand is fairness. Are you considering all the relevant viewpoints on a particular topic that exist, or have you narrowed them down to the ones you, the researcher, prefer? Are you favoring a particular explanation or particular understanding over others, without good reason? To ensure fairness, qualitative sociologists spend considerable time and effort reflecting on the biases and blind spots of their work—which is part of the reason that good qualitative work takes so long to publish. Frequently, qualitative sociologists also take steps to equalize the power dynamics in their relationships with research participants. For instance, they may try to focus their research on questions that the community being studied wants answered. They may involve participants in the data analysis process, working closely with them to arrive at a consensus on the final interpretation of the data.
Trustworthiness refers to the overall truthfulness and usefulness of the results of the research study. We can think of trustworthiness in three dimensions (credibility, dependability, and confirmability), which resemble the criteria of validity and reliability used to evaluate quantitative studies, though with important differences in emphasis.
Credibility refers to the degree to which the results are accurate and viewed as important and believable. Credibility is akin to validity, because it speaks to how closely the study reflects the reality of the people being studied. To ensure the credibility of their work, qualitative researchers sometimes run it by their research participants before publication. The idea is that the published work should make sense to the people who actually live or work in the spaces being studied—if it doesn’t jibe with their lived experiences, it’s not a credible account. Of course, it is also true that people immersed in a particular social setting may not be able to see what an outsider sees about it. More generally, seeking to make one’s work credible to the people being studied can have trade-offs, too, which is a chief reason that most journalists do not follow a similar practice in their reporting. (In their view, showing a story to sources before publication can create pressures to change their reporting and taints the objectivity they strive for in their work.) Nevertheless, qualitative sociologists should think long and hard about whether their study’s findings faithfully capture what happens in the social worlds being studied, and whether it is ultimately credible—to the study’s participants and its readers alike.
The criterion of dependability is similar, but not equivalent, to reliability. Much qualitative work is anything but reliable in the narrow way we previously described—ask the same question, get similar answers. As we have noted, qualitative research questions, hypotheses, and data collection processes routinely change during fieldwork. Qualitative research assumes that new concepts and connections will emerge from the data collection, and because of this process of exploration, respondents never get the exact same questions each time they are interviewed. Indeed, because qualitative research emphasizes the importance of context, it goes against the strength of the method to try to ask a particular question under the exact same conditions for all respondents. Can qualitative researchers achieve reliable results under such conditions? Not really. Instead, they aim for dependability. Their work can be considered dependable if they have followed the systematic research procedures and best practices for interviewing and observation that qualitative sociologists have developed over the years (we describe these methods in detail in Chapter 9: Ethnography and Chapter 10: In-Depth Interviews). Their work can also be considered dependable if they adequately documented their methodology and any changes made to it over the course of their fieldwork and analysis.
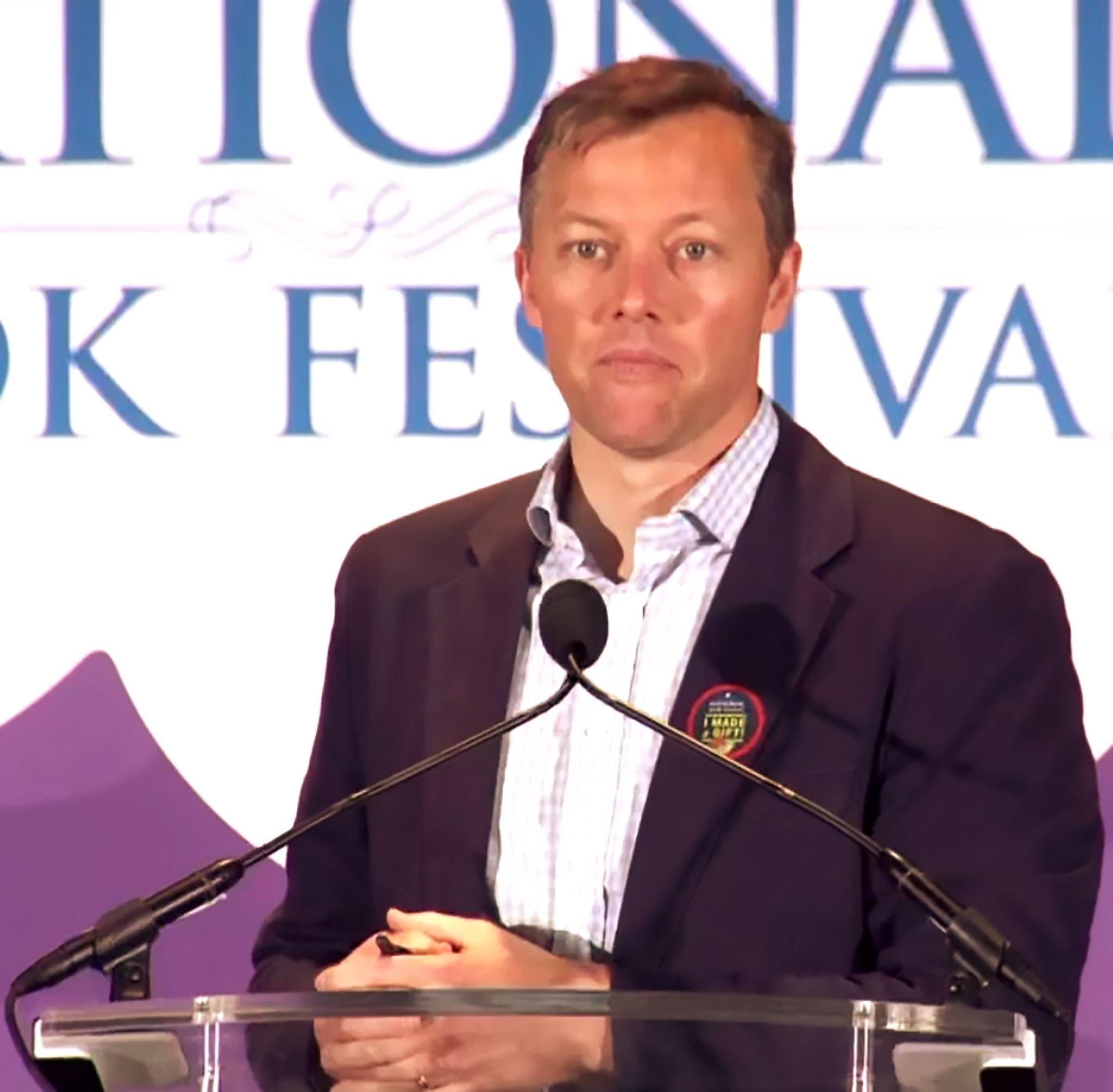
Finally, the criterion of confirmability refers to the degree to which the results reported are actually grounded in the data obtained from participants. Although it is possible that another researcher could view the same data and come up with a different analysis, confirmability ensures that a researcher’s findings have a firm basis in what participants said. Another researcher should be able to read the results of your study and trace each point made back to your data—something specific that one or more respondents shared in interviews, or something specific you observed. (This process of review is called an audit.) Any patterns observed in your data should not be exaggerated or otherwise mischaracterized in your research product.
In recent years, some public sociologists have followed journalists in hiring independent fact-checkers not just to conduct audits—ensuring that the write-up matches the collected data and its patterns—but also to confirm that every fact in their published work is accurate. For example, for his Pulitzer Prize–winning book Evicted (Desmond 2016), the sociologist Matthew Desmond took the extra step of paying for professional fact-checking of his research. Few sociologists can afford to do this, but higher levels of vetting would seem to be wise for qualitative work with any potential to spark intense public scrutiny—especially given the risks of the sorts of backlash described in Chapter 8: Ethics.
It remains to be seen whether intensive audits and fact-checking will become the future of qualitative sociology, but in the meantime, even researchers on a shoestring budget can benefit from sharing their work with colleagues—peers and professors—before publishing it. As you might expect, it’s difficult to view your own research without bias, so another set of eyes is often helpful. Careful reviewers should be able to raise helpful questions about the confirmability, credibility, and dependability of your work. Specifically, they can tell you if your work sounds plausible to them, given what exactly you are claiming and what evidence you provide to support those claims. They can point out any key flaws or omissions and push you to justify your methodological decisions more clearly and convincingly. They can examine your fieldnotes, documentation, and results to determine whether you followed proper research procedures. Obviously, any data you share with others will have to be properly anonymized to make sure you don’t violate any confidentiality agreements with your respondents, so sharing the final research product is often much easier than opening up your field notes or interview transcripts for scrutiny. That said, even just contemplating that level of transparency will often make you, the qualitative researcher, more judicious about the sorts of arguments you make based on the data you have.
Key Takeaways
- Reliability is about consistency of measurement, and validity is about accuracy of measurement.
- The three key types of reliability are test-retest, internal consistency, and inter-rater reliability.
- Five key types of validity are face, content, predictive, convergent, and discriminant validity.
- Qualitative research uses different criteria than quantitative research for assessing its quality of measurement: authenticity, trustworthiness, credibility, dependability, and confirmability.
- Research that tries to control for this confounding variable of socioeconomic status, such as work by the economist Jesse Rothstein (2004), finds that the SAT predicts little once those background characteristics are accounted for. The research also generally finds that high school grades are more effective than SAT scores in predicting college performance, although there is considerable debate over whether considering SAT scores alongside high school grades substantially improves that prediction; testing companies argue that using grades alone might worsen existing inequalities because grade inflation is more rampant in affluent areas (Kurlaender and Dykeman 2019; Watanabe 2019). ↵
- See, for example, Earl Babbie’s Practice of Social Research textbooks (2010:153). ↵
The consistency of a measure. A measure is said to be reliable if it gives the same result upon repeated applications to the same phenomenon.
The accuracy of a measure (also called construct validity). A measure is said to be valid if it accurately reflects the meaning of the concept under study.
A method of assessing the reliability of a measure by collecting data from a sample and then retesting the same sample after a period of time. If the measure is reliable, the two measurements should be consistent.
A method of assessing the reliability of a measure by examining the degree to which two or more observers (raters) agree on the measurement of one or more cases (i.e., on the values assigned to those cases).
The degree to which participants’ answers to items within a multiple-item measure are consistent. Specifically, the answers for each item in an index or scale should be correlated with each other, as they all are supposed to measure aspects of the same overall concept.
A method of assessing the validity of a measure in which the researcher evaluates whether it is plausible and logical that a given variable (specifically, the operational definition of a concept) actually measures what it intends to measure.
A method used to assess the validity of a measure where a researcher evaluates whether the measure covers all the possible meanings, domains, and dimensions of a concept.
A method of assessing the reliability of a measure by determining if it predicts future phenomena that it should be able to predict. For example, a standardized test that successfully predicts a student’s grades in college would arguably have predictive validity as a measure of academic ability.
A method used to assess the validity of a measure by comparing scores on that measure to those derived from an existing measure of the same or a similar concept. A strong correlation between the two measures is evidence that they are both measuring the same thing, and that the new measure is therefore valid in this way. (Compare to discriminant validity.)
A method of assessing the validity of a measure by comparing scores on that measure with those derived from an existing measure that, logically speaking, should be entirely unrelated. The lack of any correlation between the two measures is evidence that they are measuring different concepts. (Compare to convergent validity.)
The difference between the measured values of a variable and the true but unobserved values of that variable. The two kinds of measurement error are random error and systematic error.
A type of measurement error—present in every measurement—where unpredictable differences occur between the measured values of a variable and the true but unobserved values. (Compare to systematic error.)
A type of measurement error that occurs when a measure consistently produces incorrect data. Systematic error typically occurs in one direction, in that the measured values are consistently too high or too low compared to their true values. (Compare to random error.)
When the wording of a question encourages a person to answer in a potentially biased way.
A systematic error that may make research findings inaccurate in some way. Note that the term “bias” in this context does not just refer to the researcher’s personal biases, but to anything that causes a study’s results to fail to truthfully represent reality.
Bias that occurs when participants in a research study answer or act in particular ways to present themselves to the researcher in a more positive light.
Bias that occurs when people tend to say “yes” to whatever the researcher asks, even when doing so contradicts previous answers. (Also known as yea-saying.)
How a researcher’s personal identities and perspectives shape how they interpret reality, including what they observe over the course of their research and what conclusions they draw.
A criterion for assessing the rigor of qualitative research that focuses on the extent to which researchers capture the multiple perspectives of their research participants. Fairness is a dimension of authenticity.
A criterion used to assess the rigor of qualitative research that focuses on the extent to which researchers have considered all relevant viewpoints on a particular topic. Fairness is a dimension of the larger criterion of authenticity.
A criterion for assessing the rigor of qualitative research that speaks to the overall truthfulness and usefulness of the results of a study. There are three dimensions of trustworthiness: credibility, dependability, and confirmability.
A criterion used to assess the rigor of qualitative research that focuses on the degree to which the results are accurate and viewed as important and believable. Credibility is similar to validity in that it assesses how closely the study reflects the reality of the people being studied. It is a dimension of the larger criterion of trustworthiness.
A criterion for assessing the rigor of qualitative research that focuses on whether the researcher followed proper procedures in conducting the project. Dependability is a dimension of the larger criterion of trustworthiness.
A criterion used to assess the rigor of qualitative research that focuses on the degree to which the results reported are actually grounded in the data obtained from study participants. Confirmability is a dimension of the larger criterion of trustworthiness.
A method used to evaluate the rigor of qualitative research by tracing each point made in the published study back to the original data that was its basis.