14. Quantitative Data Analysis
Numbers pervade our lives. For one thing, we’re bombarded with them in the mass media, as seen in the following examples from news articles:
- “Incidents of white supremacist propaganda distributed across the nation jumped by more than 120% between 2018 and last year, according to the Anti-Defamation League, making 2019 the second straight year that the circulation of propaganda material has more than doubled” (Schor 2020).
- “More than 20% of black respondents were in families with [worries about their medical bills]. That compares with 13% of whites, more than 15% of those who identified as Hispanic and 7% of Asians” (Murphy 2020).
- “In the new study, among the 84 [Covid] patients who took hydroxychloroquine, 20.2% were admitted to the ICU or died within seven days of taking the drug. Among the 97 patients who did not take the drug, 22.1% went to the ICU or died. The difference was not determined to be statistically different” (Nigam and Cohen 2020).
Sociologists publish a lot of research involving quantitative data analysis. As an informed reader of this literature, you should appreciate the many ways that quantitative data can be used in sociology and learn how to interpret simple analyses. At this point, however, we don’t expect you to understand much of what is going on in the quantitative research articles you read. We also recognize how intimidating this work can be. Many students get anxious whenever math comes up, and they do their best to avoid courses that involve quantitative data analysis.
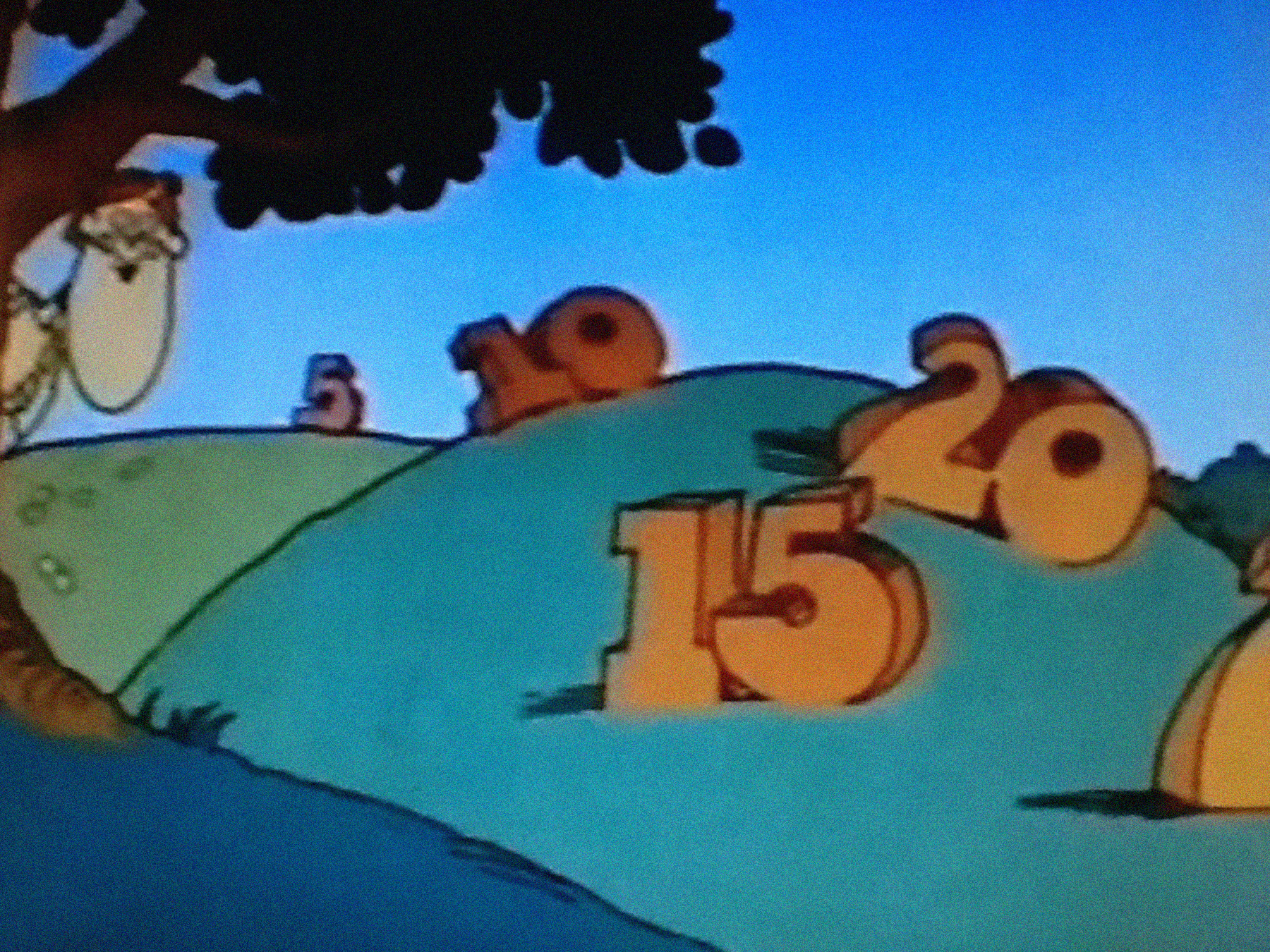
Luckily, if you’re in that group, you can relax. Our goal for this chapter is to make you sufficiently familiar with statistical analysis so that you get some useful information from quantitative work—even from more complicated analyses. You won’t need to do any calculations to understand this material. And there will be no formulas, we promise!
How do sociologists manage to do all of the mathematical calculations needed to process, say, a survey that involves data collection from hundreds or even thousands of people? The answer is that they don’t—data analysis programs do that work for them. This is another reason that you should not be afraid of quantitative data analysis even if you are math-phobic. For basic applications, the software handles most, if not all, of the numerical heavy lifting, meaning you often just need an intuitive understanding of quantitative procedures to interpret or even generate useful results.
For the rest of this chapter, we’re going to use SPSS, the Statistical Package for the Social Sciences, to walk you through quantitative data analysis. First marketed in 1968, SPSS is often available free to students in colleges and universities to use in their courses and research projects. The following chapter sections will refer to SPSS specifically, and the data will be processed in SPSS, but the discussion will apply to social scientific data analysis programs in general.
We’ll start off by talking about how you should go about either entering the data from your own survey into SPSS or finding one of thousands of existing data files available for free on the internet. Once you have data to analyze, you should generate univariate statistics (statistics examining one variable at a time) to describe your data. We’ll cover the logic behind this analysis and what the results should look like in the tables and charts you generate in SPSS. Next we’ll talk about bivariate statistics (statistics examining the relationship between two variables). We’ll go over a simple kind of bivariate analysis, crosstabulation. We’ll discuss the logic of inferential statistics—determining whether a relationship between two or more variables truly exists—and apply the chi-square test of statistical significance to our crosstabulation results. We’ll wrap up the chapter by briefly describing more advanced approaches to quantitative analysis. The goal in this chapter is to give you an intuitive understanding of when and how to apply these different statistical procedures, rather than trying to explain the math or statistical theory behind each procedure. (For that background, consult a statistics textbook.)
Statistical Analysis Programs
We find SPSS to be the easiest statistical analysis program for students to learn. It has a graphical user interface, so you don’t have to type in commands as you do with most other data analysis programs. Note, however, that social scientists use a wide range of programs, so you will inevitably come across data files in other (non-SPSS) formats, charts that look different from the standard in SPSS, and other sorts of differences that might throw you off. That said, we believe if you understand one data analysis program well, you’ll be able to at least grasp how other programs work (and remember from Chapter 2: Using Sociology in Everyday Life that employers see quantitative analysis skills as highly attractive in job candidates).
There are pros and cons to every statistical analysis program on the market. If you go the route of specializing in quantitative analysis, you should pick the one that suits you best. SPSS is commonly used in psychology, and Stata in economics. SAS is a standard at many government agencies and in the field of public health. (No particular program seems to dominate within sociology overall, though certain subfields have their favorites.) Colleges and universities often provide licenses for different data analysis programs to their students for free, just as employers do for their workers. The software licenses are very expensive and are not something that most students can or should pay for on their own.
In fact, if you are bothering to learn a different statistical analysis program than the easy-to-use SPSS, we’d highly suggest you learn R. This analysis platform, which is completely free and open-source, was created by University of Auckland researchers in 1993 and has exploded in popularity since, with a vibrant developer community that creates and freely shares modules to expand the functionality of the software. Quantitative sociologists and other researchers have adopted it in large numbers, using it for tasks ranging from basic statistical calculations to highly specialized analyses that use tailored modules. You can’t go wrong learning R, particularly given its future prospects as the go-to data analysis program. However, R (at least in its plain-vanilla form) has a steeper learning curve than SPSS, so we use the latter program in this textbook (remember, we’re trying really hard not to scare you!).
Opening chapter image credit: Karolina Grabowska, via Pexels. Adapted by Bizhan Khodabandeh.