22 13. Experimental design
Chapter outline
- What is an experiment and when should you use one? (3 minute read)
- The logic of experimental design (4 minute read)
- True experimental designs (7 minute read)
- Quasi-experimental designs (8 minute read)
- Non-experimental designs (5 minute read)
- Critical perspectives, ethics and culture (5 minute read)
Content warning: examples in this chapter contain references to non-consensual research in Western history, including experiments conducted during the Holocaust and on African Americans (section 13.6).
Learning Objectives
Learners will be able to…
- Identify the characteristics of a basic experiment
13.1 What is an experiment and when should you use one?
The first experiment I can remember using was for my fourth grade science fair. I wondered if latex- or oil-based paint would hold up to sunlight better. So, I went to the hardware store and got a few small cans of paint and two sets of wooden paint sticks. I painted one with oil-based paint and the other with latex-based paint of different colors and put them in a sunny spot in the back yard. My hypothesis was that the oil-based paint would fade the most and that more fading would happen the longer I left the paint sticks out. (I know, it’s obvious, but I was only 10.)
I checked in on the paint sticks every few days for a month and wrote down my observations. The first part of my hypothesis ended up being wrong – it was actually the latex-based paint that faded the most. But the second part was right, and the paint faded more and more over time. This is a simple example, of course – experiments get a heck of a lot more complex than this when we’re talking about real research.
Merriam-Webster defines an experiment as “an operation or procedure carried out under controlled conditions in order to discover an unknown effect or law, to test or establish a hypothesis, or to illustrate a known law.” Each of these three components of the definition will come in handy as we go through the different types of experimental design in this chapter. Most of us probably think of the physical sciences when we think of experiments, and for good reason – these experiments can be pretty flashy! But social science and psychological research follow the same scientific methods, as we’ve discussed in this book.
As the video discusses, experiments can be used in social sciences just like they can in physical sciences. It makes sense to use an experiment when you want to determine the cause of a phenomenon with as much accuracy as possible. Some types of experimental designs do this more precisely than others, as we’ll see throughout the chapter. If you’ll remember back to Chapter 11.4 and the discussion of validity, experiments are the best way to ensure internal validity, or the extent to which a change in your independent variable causes a change in your dependent variable.
Experimental designs for research projects are most appropriate when trying to uncover or test a hypothesis about the cause of a phenomenon, so they are best for explanatory research questions. As we’ll learn throughout this chapter, different circumstances are appropriate for different types of experimental designs. Each type of experimental design has advantages and disadvantages, and some are better at controlling the effect of extraneous variables – those variables and characteristics that have an effect on your dependent variable, but aren’t the primary variable whose influence you’re interested in testing. For example, in a study that tries to determine whether aspirin lowers a person’s risk of a fatal heart attack, a person’s race would likely be an extraneous variable because you primarily want to know the effect of aspirin.
In practice, many types of experimental designs can be logistically challenging and resource-intensive. As practitioners, the likelihood that we will be involved in some of the types of experimental designs discussed in this chapter is fairly low. However, it’s important to learn about these methods, even if we might not ever use them, so that we can be thoughtful consumers of research that uses experimental designs.
While we might not use all of these types of experimental designs, many of us will engage in evidence-based practice during our time as social workers. A lot of research developing evidence-based practice, which has a strong emphasis on generalizability, will use experimental designs. You’ve undoubtedly seen one or two in your literature search so far.
13.2 The logic of experimental design
Learning Objectives
- Describe causality in experimental design
- Discuss the relationship between dependent and independent variables in experiments
- Explain the links between experiments and generalizability of results
- Describe advantages and disadvantages of experimental designs
How do we know that one phenomenon causes another? The complexity of the social world in which we practice and conduct research means that causes of social problems are rarely cut and dry. Uncovering explanations for social problems is key to helping clients address them, and experimental research designs are one road to finding answers.
As you read about in Chapter 8 (and as we’ll discuss again in Chapter 15), just because two phenomena are related in some way doesn’t mean that one causes the other. Ice cream sales increase in the summer, and so does the rate of violent crime; does that mean that eating ice cream is going to make me murder someone? Obviously not, because ice cream is great. The reality of that relationship is far more complex – it could be that hot weather makes people more irritable and, at times, violent, while also making people want ice cream. More likely, though, there are other social factors not accounted for in the way we just described this relationship.
Experimental designs can help clear up at least some of this fog by allowing researchers to isolate the effect of interventions on dependent variables by controlling extraneous variables. In true experimental design (discussed in the next section) and some quasi-experimental designs, researchers accomplish this with the control group and the experimental group. (The experimental group is sometimes called the “treatment group,” but we will call it the experimental group in this chapter.) The control group does not receive the intervention you are testing (they may receive no intervention or what is known as “treatment as usual”), while the experimental group does. (You will hopefully remember our earlier discussion of control variables in Chapter 8 – conceptually, the use of the word “control” here is the same.)
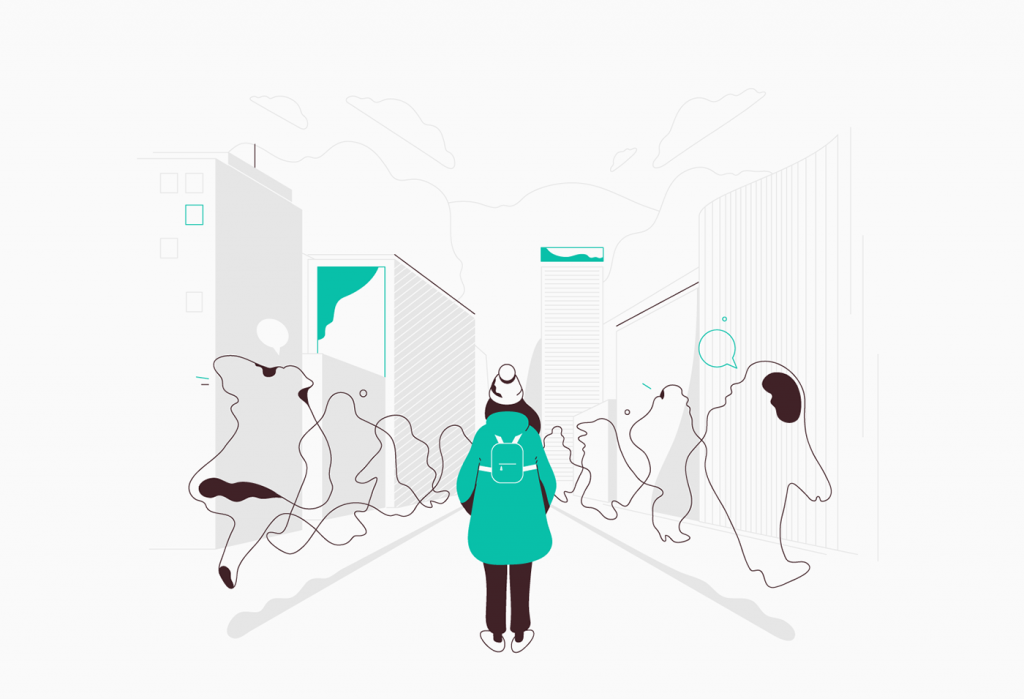
In a well-designed experiment, your control group should look almost identical to your experimental group in terms of demographics and other relevant factors. What if we want to know the effect of CBT on social anxiety, but we have learned in prior research that men tend to have a more difficult time overcoming social anxiety? We would want our control and experimental groups to have a similar gender mix because it would limit the effect of gender on our results, since ostensibly, both groups’ results would be affected by gender in the same way. If your control group has 5 women, 6 men, and 4 non-binary people, then your experimental group should be made up of roughly the same gender balance to help control for the influence of gender on the outcome of your intervention. (In reality, the groups should be similar along other dimensions, as well, and your group will likely be much larger.) The researcher will use the same outcome measures for both groups and compare them, and assuming the experiment was designed correctly, get a pretty good answer about whether the intervention had an effect on social anxiety.
You will also hear people talk about comparison groups, which are similar to control groups. The primary difference between the two is that a control group is populated using random assignment, but a comparison group is not. Random assignment entails using a random process to decide which participants are put into the control or experimental group (which participants receive an intervention and which do not). By randomly assigning participants to a group, you can reduce the effect of extraneous variables on your research because there won’t be a systematic difference between the groups.
Do not confuse random assignment with random sampling. Random sampling is a method for selecting a sample from a population, and is rarely used in psychological research. Random assignment is a method for assigning participants in a sample to the different conditions, and it is an important element of all experimental research in psychology and other related fields. Random sampling also helps a great deal with generalizability, whereas random assignment increases internal validity.
We have already learned about internal validity in Chapter 11. The use of an experimental design will bolster internal validity since it works to isolate causal relationships. As we will see in the coming sections, some types of experimental design do this more effectively than others. It’s also worth considering that true experiments, which most effectively show causality, are often difficult and expensive to implement. Although other experimental designs aren’t perfect, they still produce useful, valid evidence and may be more feasible to carry out.
Key Takeaways
- Experimental designs are useful for establishing causality, but some types of experimental design do this better than others.
- Experiments help researchers isolate the effect of the independent variable on the dependent variable by controlling for the effect of extraneous variables.
- Experiments use a control/comparison group and an experimental group to test the effects of interventions. These groups should be as similar to each other as possible in terms of demographics and other relevant factors.
- True experiments have control groups with randomly assigned participants, while other types of experiments have comparison groups to which participants are not randomly assigned.
Exercises
- Think about the research project you’ve been designing so far. How might you use a basic experiment to answer your question? If your question isn’t explanatory, try to formulate a new explanatory question and consider the usefulness of an experiment.
- Why is establishing a simple relationship between two variables not indicative of one causing the other?
13.3 True experimental design
Learning Objectives
Learners will be able to…
- Describe a true experimental design in social work research
- Understand the different types of true experimental designs
- Determine what kinds of research questions true experimental designs are suited for
- Discuss advantages and disadvantages of true experimental designs
True experimental design, often considered to be the “gold standard” in research designs, is thought of as one of the most rigorous of all research designs. In this design, one or more independent variables are manipulated by the researcher (as treatments), subjects are randomly assigned to different treatment levels (random assignment), and the results of the treatments on outcomes (dependent variables) are observed. The unique strength of experimental research is its internal validity (causality) due to its ability to link cause and effect through treatment manipulation, while controlling for the effects of extraneous variable. Sometimes the treatment level is no treatment, while other times it is simply a different treatment than that which we are trying to evaluate. For example, we might have a control group that is made up of people who will not receive any treatment for a particular condition. Or, a control group could consist of people who consent to treatment with DBT when we are testing the effectiveness of CBT.
As we discussed in the previous section, a true experiment has a control group with participants randomly assigned, and an experimental group. This is the most basic element of a true experiment. The next decision a researcher must make is when they need to gather data during their experiment. Do they take a baseline measurement and then a measurement after treatment, or just a measurement after treatment, or do they handle measurement another way? Below, we’ll discuss the three main types of true experimental designs. There are sub-types of each of these designs, but here, we just want to get you started with some of the basics.
Using a true experiment in social work research is often pretty difficult, since as I mentioned earlier, true experiments can be quite resource intensive. True experiments work best with relatively large sample sizes, and random assignment, a key criterion for a true experimental design, is hard (and unethical) to execute in practice when you have people in dire need of an intervention. Nonetheless, some of the strongest evidence bases are built on true experiments.
For the purposes of this section, let’s bring back the example of CBT for the treatment of social anxiety. We have a group of 500 individuals who have agreed to participate in our study, and we have randomly assigned them to the control and experimental groups. The folks in the experimental group will receive CBT, while the folks in the control group will receive more unstructured, basic talk therapy. These designs, as we talked about above, are best suited for explanatory research questions.
Before we get started, take a look at the table below. When explaining experimental research designs, we often use diagrams with abbreviations to visually represent the experiment. Table 13.1 starts us off by laying out what each of the abbreviations mean.
R | Randomly assigned group (control/comparison or experimental) |
O | Observation/measurement taken of dependent variable |
X | Intervention or treatment |
Xe | Experimental or new intervention |
Xi | Typical intervention/treatment as usual |
A, B, C, etc. | Denotes different groups (control/comparison and experimental) |
Pretest and post-test control group design
In pretest and post-test control group design, participants are given a pretest of some kind to measure their baseline state before their participation in an intervention. In our social anxiety experiment, we would have participants in both the experimental and control groups complete some measure of social anxiety – most likely an established scale and/or a structured interview – before they start their treatment. As part of the experiment, we would have a defined time period during which the treatment would take place (let’s say 12 weeks, just for illustration). At the end of 12 weeks, we would give both groups the same measure as a post-test.
In the diagram, RA (random assignment group A) is the experimental group and RB is the control group. O1 denotes the pre-test, Xe denotes the experimental intervention, and O2 denotes the post-test. Let’s look at this diagram another way, using the example of CBT for social anxiety that we’ve been talking about.
In a situation where the control group received treatment as usual instead of no intervention, the diagram would look this way, with Xi denoting treatment as usual (Figure 13.3).
Hopefully, these diagrams provide you a visualization of how this type of experiment establishes time order, a key component of a causal relationship. Did the change occur after the intervention? Assuming there is a change in the scores between the pretest and post-test, we would be able to say that yes, the change did occur after the intervention. Causality can’t exist if the change happened before the intervention – this would mean that something else led to the change, not our intervention.
Post-test only control group design
Post-test only control group design involves only giving participants a post-test, just like it sounds (Figure 13.4).
But why would you use this design instead of using a pretest/post-test design? One reason could be the testing effect that can happen when research participants take a pretest. In research, the testing effect refers to “measurement error related to how a test is given; the conditions of the testing, including environmental conditions; and acclimation to the test itself” (Engel & Schutt, 2017, p. 444)[1] (When we say “measurement error,” all we mean is the accuracy of the way we measure the dependent variable.) Figure 13.4 is a visualization of this type of experiment. The testing effect isn’t always bad in practice – our initial assessments might help clients identify or put into words feelings or experiences they are having when they haven’t been able to do that before. In research, however, we might want to control its effects to isolate a cleaner causal relationship between intervention and outcome.
Going back to our CBT for social anxiety example, we might be concerned that participants would learn about social anxiety symptoms by virtue of taking a pretest. They might then identify that they have those symptoms on the post-test, even though they are not new symptoms for them. That could make our intervention look less effective than it actually is.
However, without a baseline measurement establishing causality can be more difficult. If we don’t know someone’s state of mind before our intervention, how do we know our intervention did anything at all? Establishing time order is thus a little more difficult. You must balance this consideration with the benefits of this type of design.
Solomon four group design
One way we can possibly measure how much the testing effect might change the results of the experiment is with the Solomon four group design. Basically, as part of this experiment, you have two control groups and two experimental groups. The first pair of groups receives both a pretest and a post-test. The other pair of groups receives only a post-test (Figure 13.5). This design helps address the problem of establishing time order in post-test only control group designs.
For our CBT project, we would randomly assign people to four different groups instead of just two. Groups A and B would take our pretest measures and our post-test measures, and groups C and D would take only our post-test measures. We could then compare the results among these groups and see if they’re significantly different between the folks in A and B, and C and D. If they are, we may have identified some kind of testing effect, which enables us to put our results into full context. We don’t want to draw a strong causal conclusion about our intervention when we have major concerns about testing effects without trying to determine the extent of those effects.
Solomon four group designs are less common in social work research, primarily because of the logistics and resource needs involved. Nonetheless, this is an important experimental design to consider when we want to address major concerns about testing effects.
Key Takeaways
- True experimental design is best suited for explanatory research questions.
- True experiments require random assignment of participants to control and experimental groups.
- Pretest/post-test research design involves two points of measurement – one pre-intervention and one post-intervention.
- Post-test only research design involves only one point of measurement – post-intervention. It is a useful design to minimize the effect of testing effects on our results.
- Solomon four group research design involves both of the above types of designs, using 2 pairs of control and experimental groups. One group receives both a pretest and a post-test, while the other receives only a post-test. This can help uncover the influence of testing effects.
Exercises
- Think about a true experiment you might conduct for your research project. Which design would be best for your research, and why?
- What challenges or limitations might make it unrealistic (or at least very complicated!) for you to carry your true experimental design in the real-world as a student researcher?
- What hypothesis(es) would you test using this true experiment?
13.4 Quasi-experimental designs
Learning Objectives
Learners will be able to…
- Describe a quasi-experimental design in social work research
- Understand the different types of quasi-experimental designs
- Determine what kinds of research questions quasi-experimental designs are suited for
- Discuss advantages and disadvantages of quasi-experimental designs
Quasi-experimental designs are a lot more common in social work research than true experimental designs. Although quasi-experiments don’t do as good a job of giving us robust proof of causality, they still allow us to establish time order, which is a key element of causality. The prefix quasi means “resembling,” so quasi-experimental research is research that resembles experimental research, but is not true experimental research. Nonetheless, given proper research design, quasi-experiments can still provide extremely rigorous and useful results.
There are a few key differences between true experimental and quasi-experimental research. The primary difference between quasi-experimental research and true experimental research is that quasi-experimental research does not involve random assignment to control and experimental groups. Instead, we talk about comparison groups in quasi-experimental research instead. As a result, these types of experiments don’t control the effect of extraneous variables as well as a true experiment.
Quasi-experiments are most likely to be conducted in field settings in which random assignment is difficult or impossible. They are often conducted to evaluate the effectiveness of a treatment—perhaps a type of psychotherapy or an educational intervention. We’re able to eliminate some threats to internal validity, but we can’t do this as effectively as we can with a true experiment. Realistically, our CBT-social anxiety project is likely to be a quasi experiment, based on the resources and participant pool we’re likely to have available.
It’s important to note that not all quasi-experimental designs have a comparison group. There are many different kinds of quasi-experiments, but we will discuss the three main types below: nonequivalent comparison group designs, time series designs, and ex post facto comparison group designs.
Nonequivalent comparison group design
You will notice that this type of design looks extremely similar to the pretest/post-test design that we discussed in section 13.3. But instead of random assignment to control and experimental groups, researchers use other methods to construct their comparison and experimental groups. A diagram of this design will also look very similar to pretest/post-test design, but you’ll notice we’ve removed the “R” from our groups, since they are not randomly assigned (Figure 13.6).
Researchers using this design select a comparison group that’s as close as possible based on relevant factors to their experimental group. Engel and Schutt (2017)[2] identify two different selection methods:
- Individual matching: Researchers take the time to match individual cases in the experimental group to similar cases in the comparison group. It can be difficult, however, to match participants on all the variables you want to control for.
- Aggregate matching: Instead of trying to match individual participants to each other, researchers try to match the population profile of the comparison and experimental groups. For example, researchers would try to match the groups on average age, gender balance, or median income. This is a less resource-intensive matching method, but researchers have to ensure that participants aren’t choosing which group (comparison or experimental) they are a part of.
As we’ve already talked about, this kind of design provides weaker evidence that the intervention itself leads to a change in outcome. Nonetheless, we are still able to establish time order using this method, and can thereby show an association between the intervention and the outcome. Like true experimental designs, this type of quasi-experimental design is useful for explanatory research questions.
What might this look like in a practice setting? Let’s say you’re working at an agency that provides CBT and other types of interventions, and you have identified a group of clients who are seeking help for social anxiety, as in our earlier example. Once you’ve obtained consent from your clients, you can create a comparison group using one of the matching methods we just discussed. If the group is small, you might match using individual matching, but if it’s larger, you’ll probably sort people by demographics to try to get similar population profiles. (You can do aggregate matching more easily when your agency has some kind of electronic records or database, but it’s still possible to do manually.)
Time series design
Another type of quasi-experimental design is a time series design. Unlike other types of experimental design, time series designs do not have a comparison group. A time series is a set of measurements taken at intervals over a period of time (Figure 13.7). Proper time series design should include at least three pre- and post-intervention measurement points. While there are a few types of time series designs, we’re going to focus on the most common: interrupted time series design.
But why use this method? Here’s an example. Let’s think about elementary student behavior throughout the school year. As anyone with children or who is a teacher knows, kids get very excited and animated around holidays, days off, or even just on a Friday afternoon. This fact might mean that around those times of year, there are more reports of disruptive behavior in classrooms. What if we took our one and only measurement in mid-December? It’s possible we’d see a higher-than-average rate of disruptive behavior reports, which could bias our results if our next measurement is around a time of year students are in a different, less excitable frame of mind. When we take multiple measurements throughout the first half of the school year, we can establish a more accurate baseline for the rate of these reports by looking at the trend over time.
We may want to test the effect of extended recess times in elementary school on reports of disruptive behavior in classrooms. When students come back after the winter break, the school extends recess by 10 minutes each day (the intervention), and the researchers start tracking the monthly reports of disruptive behavior again. These reports could be subject to the same fluctuations as the pre-intervention reports, and so we once again take multiple measurements over time to try to control for those fluctuations.
This method improves the extent to which we can establish causality because we are accounting for a major extraneous variable in the equation – the passage of time. On its own, it does not allow us to account for other extraneous variables, but it does establish time order and association between the intervention and the trend in reports of disruptive behavior. Finding a stable condition before the treatment that changes after the treatment is evidence for causality between treatment and outcome.
Ex post facto comparison group design
Ex post facto (Latin for “after the fact”) designs are extremely similar to nonequivalent comparison group designs. There are still comparison and experimental groups, pretest and post-test measurements, and an intervention. But in ex post facto designs, participants are assigned to the comparison and experimental groups once the intervention has already happened. This type of design often occurs when interventions are already up and running at an agency and the agency wants to assess effectiveness based on people who have already completed treatment.
In most clinical agency environments, social workers conduct both initial and exit assessments, so there are usually some kind of pretest and post-test measures available. We also typically collect demographic information about our clients, which could allow us to try to use some kind of matching to construct comparison and experimental groups.
In terms of internal validity and establishing causality, ex post facto designs are a bit of a mixed bag. The ability to establish causality depends partially on the ability to construct comparison and experimental groups that are demographically similar so we can control for these extraneous variables.
Conclusion
Quasi-experimental designs are common in social work intervention research because, when designed correctly, they balance the intense resource needs of true experiments with the realities of research in practice. They still offer researchers tools to gather robust evidence about whether interventions are having positive effects for clients.
Key Takeaways
- Quasi-experimental designs are similar to true experiments, but do not require random assignment to experimental and control groups.
- In quasi-experimental projects, the group not receiving the treatment is called the comparison group, not the control group.
- Nonequivalent comparison group design is nearly identical to pretest/post-test experimental design, but participants are not randomly assigned to the experimental and control groups. As a result, this design provides slightly less robust evidence for causality.
- Nonequivalent groups can be constructed by individual matching or aggregate matching.
- Time series design does not have a control or experimental group, and instead compares the condition of participants before and after the intervention by measuring relevant factors at multiple points in time. This allows researchers to mitigate the error introduced by the passage of time.
- Ex post facto comparison group designs are also similar to true experiments, but experimental and comparison groups are constructed after the intervention is over. This makes it more difficult to control for the effect of extraneous variables, but still provides useful evidence for causality because it maintains the time order of the experiment.
Exercises
- Think back to the experiment you considered for your research project in Section 13.3. Now that you know more about quasi-experimental designs, do you still think it’s a true experiment? Why or why not?
- What should you consider when deciding whether an experimental or quasi-experimental design would be more feasible or fit your research question better?
13.5 Non-experimental designs
Learning Objectives
Learners will be able to…
- Describe non-experimental designs in social work research
- Discuss how non-experimental research differs from true and quasi-experimental research
- Demonstrate an understanding the different types of non-experimental designs
- Determine what kinds of research questions non-experimental designs are suited for
- Discuss advantages and disadvantages of non-experimental designs
The previous sections have laid out the basics of some rigorous approaches to establish that an intervention is responsible for changes we observe in research participants. This type of evidence is extremely important to build an evidence base for social work interventions, but it’s not the only type of evidence to consider. We will discuss qualitative methods, which provide us with rich, contextual information, in Part 4 of this text. The designs we’ll talk about in this section are sometimes used in qualitative research, but in keeping with our discussion of experimental design so far, we’re going to stay in the quantitative research realm for now. Non-experimental is also often a stepping stone for more rigorous experimental design in the future, as it can help test the feasibility of your research.
In general, non-experimental designs do not strongly support causality and don’t address threats to internal validity. However, that’s not really what they’re intended for. Non-experimental designs are useful for a few different types of research, including explanatory questions in program evaluation. Certain types of non-experimental design are also helpful for researchers when they are trying to develop a new assessment or scale. Other times, researchers or agency staff did not get a chance to gather any assessment information before an intervention began, so a pretest/post-test design is not possible.
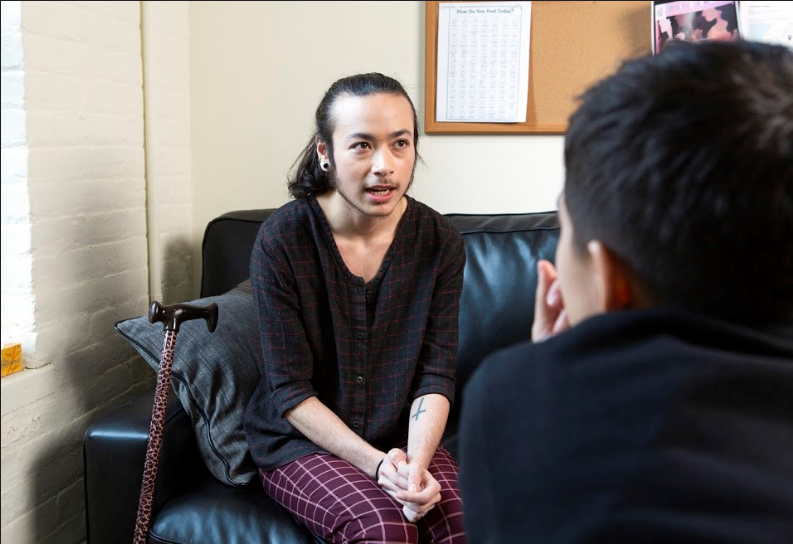
A significant benefit of these types of designs is that they’re pretty easy to execute in a practice or agency setting. They don’t require a comparison or control group, and as Engel and Schutt (2017)[3] point out, they “flow from a typical practice model of assessment, intervention, and evaluating the impact of the intervention” (p. 177). Thus, these designs are fairly intuitive for social workers, even when they aren’t expert researchers. Below, we will go into some detail about the different types of non-experimental design.
One group pretest/post-test design
Also known as a before-after one-group design, this type of research design does not have a comparison group and everyone who participates in the research receives the intervention (Figure 13.8). This is a common type of design in program evaluation in the practice world. Controlling for extraneous variables is difficult or impossible in this design, but given that it is still possible to establish some measure of time order, it does provide weak support for causality.
Imagine, for example, a researcher who is interested in the effectiveness of an anti-drug education program on elementary school students’ attitudes toward illegal drugs. The researcher could assess students’ attitudes about illegal drugs (O1), implement the anti-drug program (X), and then immediately after the program ends, the researcher could once again measure students’ attitudes toward illegal drugs (O2). You can see how this would be relatively simple to do in practice, and have probably been involved in this type of research design yourself, even if informally. But hopefully, you can also see that this design would not provide us with much evidence for causality because we have no way of controlling for the effect of extraneous variables. A lot of things could have affected any change in students’ attitudes – maybe girls already had different attitudes about illegal drugs than children of other genders, and when we look at the class’s results as a whole, we couldn’t account for that influence using this design.
All of that doesn’t mean these results aren’t useful, however. If we find that children’s attitudes didn’t change at all after the drug education program, then we need to think seriously about how to make it more effective or whether we should be using it at all. (This immediate, practical application of our results highlights a key difference between program evaluation and research, which we will discuss in Chapter 23.)
After-only design
As the name suggests, this type of non-experimental design involves measurement only after an intervention. There is no comparison or control group, and everyone receives the intervention. I have seen this design repeatedly in my time as a program evaluation consultant for nonprofit organizations, because often these organizations realize too late that they would like to or need to have some sort of measure of what effect their programs are having.
Because there is no pretest and no comparison group, this design is not useful for supporting causality since we can’t establish the time order and we can’t control for extraneous variables. However, that doesn’t mean it’s not useful at all! Sometimes, agencies need to gather information about how their programs are functioning. A classic example of this design is satisfaction surveys – realistically, these can only be administered after a program or intervention. Questions regarding satisfaction, ease of use or engagement, or other questions that don’t involve comparisons are best suited for this type of design.
Static-group design
A final type of non-experimental research is the static-group design. In this type of research, there are both comparison and experimental groups, which are not randomly assigned. There is no pretest, only a post-test, and the comparison group has to be constructed by the researcher. Sometimes, researchers will use matching techniques to construct the groups, but often, the groups are constructed by convenience of who is being served at the agency.
Conclusions
Non-experimental research designs are easy to execute in practice, but we must be cautious about drawing causal conclusions from the results. A positive result may still suggest that we should continue using a particular intervention (and no result or a negative result should make us reconsider whether we should use that intervention at all). You have likely seen non-experimental research in your daily life or at your agency, and knowing the basics of how to structure such a project will help you ensure you are providing clients with the best care possible.
Key Takeaways
Type your key takeaways here.
- Non-experimental designs are useful for describing phenomena, but cannot demonstrate causality.
- After-only designs are often used in agency and practice settings because practitioners are often not able to set up pre-test/post-test designs.
- Non-experimental designs are useful for explanatory questions in program evaluation and are helpful for researchers when they are trying to develop a new assessment or scale.
- Non-experimental designs are well-suited to qualitative methods.
Exercises
- If you were to use a non-experimental design for your research project, which would you choose? Why?
- Have you conducted non-experimental research in your practice or professional life? Which type of non-experimental design was it?
13.6 Critical perspectives, ethics and cultural considerations in experimental research
Learning Objectives
Learners will be able to…
- Describe critiques of experimental design
- Identify ethical issues in the design and execution of experiments
- Identify cultural considerations in experimental design
As I said at the outset, experiments, and especially true experiments, have long been seen as the gold standard to gather scientific evidence. When it comes to research in the biomedical field and other physical sciences, true experiments are subject to far less nuance than experiments in the social world. This doesn’t mean they are easier – just subject to different forces. However, as a society, we have placed the most value on quantitative evidence obtained through empirical observation and especially experimentation.
Major critiques of experimental designs tend to focus on true experiments, especially randomized controlled trials (RCTs), but many of these critiques can be applied to quasi-experimental designs, too. Some researchers, even in the biomedical sciences, question the view that RCTs are inherently superior to other types of quantitative research designs. RCTs are far less flexible and have much more stringent requirements than other types of research. One seemingly small issue, like incorrect information about a research participant, can derail an entire RCT. RCTs also cost a great deal of money to implement and don’t reflect “real world” conditions. The cost of true experimental research or RCTs also means that some communities are unlikely to ever have access to these research methods. It is then easy for people to dismiss their research findings because their methods are seen as “not rigorous.”
Obviously, controlling outside influences is important for researchers to draw strong conclusions, but what if those outside influences are actually important for how an intervention works? Are we missing really important information by focusing solely on control in our research? Is a treatment going to work the same for white women as it does for indigenous women? With the myriad effects of our societal structures, you should be very careful ever assuming this will be the case. This doesn’t mean that cultural differences will negate the effect of an intervention; instead, it means that you should remember to practice cultural humility implementing all interventions, even when we “know” they work.
How we build evidence through experimental research reveals a lot about our values and biases, and historically, much experimental research has been conducted on white people, and especially white men.[4] This makes sense when we consider the extent to which the sciences and academia have historically been dominated by white patriarchy. This is especially important for marginalized groups that have long been ignored in research literature, meaning they have also been ignored in the development of interventions and treatments that are accepted as “effective.” There are examples of marginalized groups being experimented on without their consent, like the Tuskegee Experiment or Nazi experiments on Jewish people during World War II. We cannot ignore the collective consciousness situations like this can create about experimental research for marginalized groups.
None of this is to say that experimental research is inherently bad or that you shouldn’t use it. Quite the opposite – use it when you can, because there are a lot of benefits, as we learned throughout this chapter. As a social work researcher, you are uniquely positioned to conduct experimental research while applying social work values and ethics to the process and be a leader for others to conduct research in the same framework. It can conflict with our professional ethics, especially respect for persons and beneficence, if we do not engage in experimental research with our eyes wide open. We also have the benefit of a great deal of practice knowledge that researchers in other fields have not had the opportunity to get. As with all your research, always be sure you are fully exploring the limitations of the research.
Key Takeaways
- While true experimental research gathers strong evidence, it can also be inflexible, expensive, and overly simplistic in terms of important social forces that affect the resources.
- Marginalized communities’ past experiences with experimental research can affect how they respond to research participation.
- Social work researchers should use both their values and ethics, and their practice experiences, to inform research and push other researchers to do the same.
Exercises
- Think back to the true experiment you sketched out in the exercises for Section 13.3. Are there cultural or historical considerations you hadn’t thought of with your participant group? What are they? Does this change the type of experiment you would want to do?
- How can you as a social work researcher encourage researchers in other fields to consider social work ethics and values in their experimental research?
- Engel, R. & Schutt, R. (2016). The practice of research in social work. Thousand Oaks, CA: SAGE Publications, Inc. ↵
- Engel, R. & Schutt, R. (2016). The practice of research in social work. Thousand Oaks, CA: SAGE Publications, Inc. ↵
- Engel, R. & Schutt, R. (2016). The practice of research in social work. Thousand Oaks, CA: SAGE Publications, Inc. ↵
- Sullivan, G. M. (2011). Getting off the “gold standard”: Randomized controlled trials and education research. Journal of Graduate Medical Education, 3(3), 285-289. ↵
an operation or procedure carried out under controlled conditions in order to discover an unknown effect or law, to test or establish a hypothesis, or to illustrate a known law.
explains why particular phenomena work in the way that they do; answers “why” questions
variables and characteristics that have an effect on your outcome, but aren't the primary variable whose influence you're interested in testing.
using a random process to decide which participants are tested in which conditions
Ability to say that one variable "causes" something to happen to another variable. Very important to assess when thinking about studies that examine causation such as experimental or quasi-experimental designs.
a type of experimental design in which participants are randomly assigned to control and experimental groups, one group receives an intervention, and both groups receive pre- and post-test assessments
an experimental design in which participants are randomly assigned to control and treatment groups, one group receives an intervention, and both groups receive only a post-test assessment
a subtype of experimental design that is similar to a true experiment, but does not have randomly assigned control and treatment groups
In nonequivalent comparison group designs, the process by which researchers match individual cases in the experimental group to similar cases in the comparison group.
In nonequivalent comparison group designs, the process in which researchers match the population profile of the comparison and experimental groups.
Research that involves the use of data that represents human expression through words, pictures, movies, performance and other artifacts.